Sztuczna inteligencja jako sojusznik w walce z cukrzycą: nowoczesne strategie leczenia
Słowa kluczowe:
AI, cukrzyca, leczenie, technologiaStreszczenie
Artykuł skupia się na omówieniu roli sztucznej inteligencji (ang. Artificial Intelligence - AI) jako innowacyjnego sojusznika w dziedzinie medycyny, szczególnie w kontekście walki z cukrzycą (ang. Diabetes Mellitus - DM). Nowoczesne strategie leczenia, które wykorzystują zaawansowane technologie oparte na AI do doskonalenia diagnostyki, personalizacji terapii oraz monitorowania pacjentów z cukrzycą są coraz bardziej cenione. Artykuł przedstawia różnorodne aspekty zastosowania AI oraz podaje przykłady systemów i algorytmów, które mogą zostać wykorzystane do samodzielnego leczenia i kontroli cukrzycy. Systemy monitorowania zdrowia oparte na technologii noszonych urządzeń integrujących dane z czujników AI w celu ścisłego monitorowania poziomu glukozy, aktywności fizycznej i innych parametrów również wiele wnoszą do nowego, rozwijającego się podejścia do leczenia cukrzycy. Te zaawansowane strategie w praktyce klinicznej podkreślają ogromny potencjał AI do poprawy skuteczności leczenia DM, minimalizacji ryzyka powikłań oraz zwiększenia jakości życia pacjentów. Konieczność etycznego i bezpiecznego wykorzystania tych technologii, a także dalszy rozwój badań w celu doskonalenia narzędzi opartych na AI w obszarze medycyny pozostaje ważną kwestią, o której stale należy pamiętać .
Bibliografia
James SL, Abate D, Abate KH, et al. Global, regional, and national incidence, prevalence, and years lived with disability for 354 diseases and injuries for 195 countries and territories, 1990–2017: a systematic analysis for the Global Burden of Disease Study 2017. The Lancet. 2018;392(10159):1789-1858. doi:10.1016/s0140-6736(18)32279-7
Vermeulen R, Schymanski EL, Barabási AL, Miller GW. The exposome and health: Where chemistry meets biology. Science. 2020;367(6476):392-396. doi:10.1126/science.aay3164
Huang J, Yeung AM, Armstrong DG, et al. Artificial Intelligence for Predicting and Diagnosing Complications of Diabetes. J Diabetes Sci Technol. 2022;17(1):224-238. doi:10.1177/19322968221124583
Sriram RD, Reddy SSK. Artificial Intelligence and Digital Tools. Clinics in Geriatric Medicine. 2020;36(3):513-525. doi:10.1016/j.cger.2020.04.009
Tahir F, Farhan M. Exploring the progress of artificial intelligence in managing type 2 diabetes mellitus: a comprehensive review of present innovations and anticipated challenges ahead. Front Clin Diabetes Healthc. 2023;4. doi:10.3389/fcdhc.2023.1316111
The Lancet. Diabetes: a defining disease of the 21st century. The Lancet. 2023;401(10394):2087. doi:10.1016/s0140-6736(23)01296-5
Hollis KF, Soualmia LF, Séroussi B. Artificial Intelligence in Health Informatics: Hype or Reality? Yearb Med Inform. 2019;28(01):003-004. doi:10.1055/s-0039-1677951
Contreras I, Vehi J. Artificial Intelligence for Diabetes Management and Decision Support: Literature Review. J Med Internet Res. 2018;20(5):e10775. doi:10.2196/10775
Guan Z, Li H, Liu R, et al. Artificial intelligence in diabetes management: Advancements, opportunities, and challenges. Cell Reports Medicine. 2023;4(10):101213. doi:10.1016/j.xcrm.2023.101213
Cai C, Liu Y, Li Y, et al. Effectiveness of quality of care for patients with type 2 diabetes in China: findings from the Shanghai Integration Model (SIM). Front Med. 2021;16(1):126-138. doi:10.1007/s11684-021-0897-7
Nomura A, Noguchi M, Kometani M, Furukawa K, Yoneda T. Artificial Intelligence in Current Diabetes Management and Prediction. Curr Diab Rep. 2021;21(12). doi:10.1007/s11892-021-01423-2
Ashrafzadeh S, Hamdy O. Patient-Driven Diabetes Care of the Future in the Technology Era. Cell Metabolism. 2019;29(3):564-575. doi:10.1016/j.cmet.2018.09.005
Subramanian M, Wojtusciszyn A, Favre L, et al. Precision medicine in the era of artificial intelligence: implications in chronic disease management. J Transl Med. 2020;18(1). doi:10.1186/s12967-020-02658-5
Vineis P, Chadeau-Hyam M, Gmuender H, et al. The exposome in practice: Design of the EXPOsOMICS project. International Journal of Hygiene and Environmental Health. 2017;220(2):142-151. doi:10.1016/j.ijheh.2016.08.001
Tran KA, Kondrashova O, Bradley A, Williams ED, Pearson JV, Waddell N. Deep learning in cancer diagnosis, prognosis and treatment selection. Genome Med. 2021;13(1). doi:10.1186/s13073-021-00968-x
Reyna MA, Nsoesie EO, Clifford GD. Rethinking Algorithm Performance Metrics for Artificial Intelligence in Diagnostic Medicine. JAMA. 2022;328(4):329. doi:10.1001/jama.2022.10561
Zhang Z, Yang L, Han W, et al. Machine Learning Prediction Models for Gestational Diabetes Mellitus: Meta-analysis. J Med Internet Res. 2022;24(3):e26634. doi:10.2196/26634
Ahmed A, Aziz S, Abd-alrazaq A, Farooq F, Sheikh J. Overview of Artificial Intelligence–Driven Wearable Devices for Diabetes: Scoping Review. J Med Internet Res. 2022;24(8):e36010. doi:10.2196/36010
Prabha A, Yadav J, Rani A, Singh V. Non-invasive Diabetes Mellitus Detection System using Machine Learning Techniques. 2021 11th International Conference on Cloud Computing, Data Science & Engineering (Confluence). Published online January 28, 2021. doi:10.1109/confluence51648.2021.9377138
Naveed I, Kaleem MF, Keshavjee K, Guergachi A. Artificial intelligence with temporal features outperforms machine learning in predicting diabetes. Pani D, ed. PLOS Digit Health. 2023;2(10):e0000354. doi:10.1371/journal.pdig.0000354
Calderaro J, Seraphin TP, Luedde T, Simon TG. Artificial intelligence for the prevention and clinical management of hepatocellular carcinoma. Journal of Hepatology. 2022;76(6):1348-1361. doi:10.1016/j.jhep.2022.01.014
Ellahham S. Artificial Intelligence: The Future for Diabetes Care. The American Journal of Medicine. 2020;133(8):895-900. doi:10.1016/j.amjmed.2020.03.033
Xie P, Li Y, Deng B, et al. An explainable machine learning model for predicting in‐hospital amputation rate of patients with diabetic foot ulcer. International Wound Journal. 2021;19(4):910-918. doi:10.1111/iwj.13691
Zubarev V. Machine learning for everyone. https://vas3k.com/blog/machine_learning/?fbclid=IwAR0f5ceCQC6v6TXg21rNdU9cvAKxNXmRtuGIqtBpIAHwsSUrFtnJpJD9nW8
Topol EJ. High-performance medicine: the convergence of human and artificial intelligence. Nat Med. 2019;25(1):44-56. doi:10.1038/s41591-018-0300-7
Rigla M, García-Sáez G, Pons B, Hernando ME. Artificial Intelligence Methodologies and Their Application to Diabetes. J Diabetes Sci Technol. 2017;12(2):303-310. doi:10.1177/1932296817710475
van Assen M, Lee SJ, De Cecco CN. Artificial intelligence from A to Z: From neural network to legal framework. European Journal of Radiology. 2020;129:109083. doi:10.1016/j.ejrad.2020.109083
Williams DM, Jones H, Stephens JW. Personalized Type 2 Diabetes Management: An Update on Recent Advances and Recommendations. DMSO. 2022;Volume 15:281-295. doi:10.2147/dmso.s331654
Facilitating Behavior Change and Well-being to Improve Health Outcomes:Standards of Medical Care in Diabetes—2020. Diabetes Care. 2019;43(Supplement_1):S48-S65. doi:10.2337/dc20-s005
Lee EY, Yun JS, Cha SA, et al. Personalized Type 2 Diabetes Management Using a Mobile Application Integrated with Electronic Medical Records: An Ongoing Randomized Controlled Trial. IJERPH. 2021;18(10):5300. doi:10.3390/ijerph18105300
Ahmed M, Zaghloul I, Abd El Fattah M. A Survey on Personalization of Diabetes Treatment using Artificial Intelligence Techniques. Benha Journal of Applied Sciences. 2023;8(5):229-236. doi:10.21608/bjas.2023.202990.1140
Ye Y, Xiong Y, Zhou Q, Wu J, Li X, Xiao X. Comparison of Machine Learning Methods and Conventional Logistic Regressions for Predicting Gestational Diabetes Using Routine Clinical Data: A Retrospective Cohort Study. Journal of Diabetes Research. 2020;2020:1-10. doi:10.1155/2020/4168340
Mendes-Soares H, Raveh-Sadka T, Azulay S, et al. Assessment of a Personalized Approach to Predicting Postprandial Glycemic Responses to Food Among Individuals Without Diabetes. JAMA Netw Open. 2019;2(2):e188102. doi:10.1001/jamanetworkopen.2018.8102
Wu YT, Zhang CJ, Mol BW, et al. Early Prediction of Gestational Diabetes Mellitus in the Chinese Population via Advanced Machine Learning. The Journal of Clinical Endocrinology & Metabolism. 2020;106(3):e1191-e1205. doi:10.1210/clinem/dgaa899
Wu Y, Ma S, Wang Y, et al. A risk prediction model of gestational diabetes mellitus before 16 gestational weeks in Chinese pregnant women. Diabetes Research and Clinical Practice. 2021;179:109001. doi:10.1016/j.diabres.2021.109001
Abràmoff MD, Lavin PT, Birch M, Shah N, Folk JC. Pivotal trial of an autonomous AI-based diagnostic system for detection of diabetic retinopathy in primary care offices. npj Digital Med. 2018;1(1). doi:10.1038/s41746-018-0040-6
Jia W, Fisher EB. Application and prospect of artificial intellingence in diabetes care. Medical Review. 2023;3(1):102-104. doi:10.1515/mr-2022-0039
Shan R, Sarkar S, Martin SS. Digital health technology and mobile devices for the management of diabetes mellitus: state of the art. Diabetologia. 2019;62(6):877-887. doi:10.1007/s00125-019-4864-7
Tang PY, Duni J, Peeples MM, et al. Complementarity of Digital Health and Peer Support: “This Is What’s Coming.” Front Clin Diabetes Healthc. 2021;2. doi:10.3389/fcdhc.2021.646963
Zhang L, He X, Shen Y, et al. Effectiveness of Smartphone App–Based Interactive Management on Glycemic Control in Chinese Patients With Poorly Controlled Diabetes: Randomized Controlled Trial. J Med Internet Res. 2019;21(12):e15401. doi:10.2196/15401
Chen S, Lu J, Peng D, et al. Effect of a Mobile Health Technology–Based Diabetes Education Program on Glucose Control in Patients With Type 2 Diabetes Initiating Premixed Insulin: A Prospective, Multicenter, Observational Study. Diabetes Care. 2022;46(1):e6-e7. doi:10.2337/dc22-0510
Andrès E et al. Telemonitoring in diabetes: evolution of concepts and technologies, with a focus on results of the more recent studies. JMedLife. 2019;12(3):203-214. doi:10.25122/jml-2019-0006
Ye J, Wu Y, Yang S, et al. The global, regional and national burden of type 2 diabetes mellitus in the past, present and future: a systematic analysis of the Global Burden of Disease Study 2019. Front Endocrinol. 2023;14. doi:10.3389/fendo.2023.1192629 36
Oh SH, Park J, Lee SJ, Kang S, Mo J. Reinforcement learning-based expanded personalized diabetes treatment recommendation using South Korean electronic health records. Expert Systems with Applications. 2022;206:117932. doi:10.1016/j.eswa.2022.117932
Villena Gonzales W, Mobashsher A, Abbosh A. The Progress of Glucose Monitoring—A Review of Invasive to Minimally and Non-Invasive Techniques, Devices and Sensors. Sensors. 2019;19(4):800. doi:10.3390/s19040800
Gulshan V, Peng L, Coram M, et al. Development and Validation of a Deep Learning Algorithm for Detection of Diabetic Retinopathy in Retinal Fundus Photographs. JAMA. 2016;316(22):2402. doi:10.1001/jama.2016.17216
Nimri R, Battelino T, Laffel LM, et al. Insulin dose optimization using an automated artificial intelligence-based decision support system in youths with type 1 diabetes. Nat Med. 2020;26(9):1380-1384. doi:10.1038/s41591-020-1045-7
Ravaut M, Harish V, Sadeghi H, et al. Development and Validation of a Machine Learning Model Using Administrative Health Data to Predict Onset of Type 2 Diabetes. JAMA Netw Open. 2021;4(5):e2111315. doi:10.1001/jamanetworkopen.2021.11315
Coronato A, Naeem M, De Pietro G, Paragliola G. Reinforcement learning for intelligent healthcare applications: A survey. Artificial Intelligence in Medicine. 2020;109:101964. doi:10.1016/j.artmed.2020.101964
Gianfrancesco MA, Tamang S, Yazdany J, Schmajuk G. Potential Biases in Machine Learning Algorithms Using Electronic Health Record Data. JAMA Intern Med. 2018;178(11):1544. doi:10.1001/jamainternmed.2018.3763
Gomez-Carmona O, Casado-Mansilla D, Lopez-de-Ipina D, Garcia-Zubia J. Optimizing Computational Resources for Edge Intelligence Through Model Cascade Strategies. IEEE Internet Things J. 2022;9(10):7404-7417. doi:10.1109/jiot.2021.3118845
Classification and Diagnosis of Diabetes:Standards of Medical Care in Diabetes—2021. Diabetes Care. 2020;44(Supplement_1):S15-S33. doi:10.2337/dc21-s002
Abhari S, Niakan Kalhori SR, Ebrahimi M, Hasannejadasl H, Garavand A. Artificial Intelligence Applications in Type 2 Diabetes Mellitus Care: Focus on Machine Learning Methods. Healthc Inform Res. 2019;25(4):248. doi:10.4258/hir.2019.25.4.248
Li J, Yuan P, Hu X, et al. A tongue features fusion approach to predicting prediabetes and diabetes with machine learning. Journal of Biomedical Informatics. 2021;115:103693. doi:10.1016/j.jbi.2021.103693
Maniruzzaman Md, Kumar N, Menhazul Abedin Md, et al. Comparative approaches for classification of diabetes mellitus data: Machine learning paradigm. Computer Methods and Programs in Biomedicine. 2017;152:23-34. doi:10.1016/j.cmpb.2017.09.004
Abbasi A, Peelen LM, Corpeleijn E, et al. Prediction models for risk of developing type 2 diabetes: systematic literature search and independent external validation study. BMJ. 2012;345(sep18 2):e5900-e5900. doi:10.1136/bmj.e5900
Lai H, Huang H, Keshavjee K, Guergachi A, Gao X. Predictive models for diabetes mellitus using machine learning techniques. BMC Endocr Disord. 2019;19(1). doi:10.1186/s12902-019-0436-6
Kopitar L, Kocbek P, Cilar L, Sheikh A, Stiglic G. Early detection of type 2 diabetes mellitus using machine learning-based prediction models. Sci Rep. 2020;10(1). doi:10.1038/s41598-020-68771-z
Zhang L, Wang Y, Niu M, Wang C, Wang Z. Machine learning for characterizing risk of type 2 diabetes mellitus in a rural Chinese population: the Henan Rural Cohort Study. Sci Rep. 2020;10(1). doi:10.1038/s41598-020-61123-x
Nomura A, Yamamoto S, Hayakawa Y, et al. SAT-LB121 Development of a Machine-Learning Method for Predicting New Onset of Diabetes Mellitus: A Retrospective Analysis of 509,153 Annual Specific Health Checkup Records. Journal of the Endocrine Society. 2020;4(Supplement_1). doi:10.1210/jendso/bvaa046.2194
Zhou W, Sailani MR, Contrepois K, et al. Longitudinal multi-omics of host–microbe dynamics in prediabetes. Nature. 2019;569(7758):663-671. doi:10.1038/s41586-019-1236-x
Ho D, Quake SR, McCabe ERB, et al. Enabling Technologies for Personalized and Precision Medicine. Trends in Biotechnology. 2020;38(5):497-518. doi:10.1016/j.tibtech.2019.12.021
Sattler F, Muller KR, Samek W. Clustered Federated Learning: Model-Agnostic Distributed Multitask Optimization Under Privacy Constraints. IEEE Trans Neural Netw Learning Syst. 2021;32(8):3710-3722. doi:10.1109/tnnls.2020.3015958
Yuan B, Li J. The Policy Effect of the General Data Protection Regulation (GDPR) on the Digital Public Health Sector in the European Union: An Empirical Investigation. IJERPH. 2019;16(6):1070. doi:10.3390/ijerph16061070
Gerke S, Minssen T, Cohen G. Ethical and legal challenges of artificial intelligence-driven healthcare. Artificial Intelligence in Healthcare. Published online 2020:295-336. doi:10.1016/b978-0-12-818438-7.00012-5
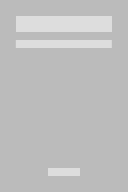
Zapowiedzi
Licencja

Utwór dostępny jest na licencji Creative Commons Uznanie autorstwa – Użycie niekomercyjne – Bez utworów zależnych 4.0 Międzynarodowe.