Wykorzystanie sztucznej inteligencji w wykrywaniu czerniaka skóry: aktualne zastosowania, wyzwania i perspektywy
Słowa kluczowe:
sztuczna inteligencja, ai, czerniak, dermatologia, diagnostyka, uczenie maszynowe, głębokie uczenieStreszczenie
W ostatnich dekadach, rozwój i zastosowanie sztucznej inteligencji (SI) w dziedzinie medycyny, a w szczególności w diagnostyce czerniaka, przeobraziło podejście do wczesnego rozpoznawania i leczenia tego agresywnego rodzaju raka skóry. Niniejsza praca koncentruje się na aktualnych zastosowaniach, wyzwaniach i przyszłych perspektywach włączenia technologii SI do diagnostyki czerniaka, z naciskiem na zwiększenie dokładności, szybkości oraz możliwości prognostycznych w ocenie ryzyka czerniaka. Omówione zostały kluczowe metody, takie jak algorytmy uczenia maszynowego i głębokiego uczenia, które wykorzystują obrazy dermatoskopowe i histopatologiczne w celu identyfikacji cech charakterystycznych dla czerniaka. Zwrócono również uwagę na znaczenie dużej, zróżnicowanej i dobrze zanotowanej bazy danych obrazowych w skutecznym treningu modeli AI, a także wyzwania takie jak integracja tych technologii w praktyce klinicznej, zapewnienie prywatności danych, oraz potrzeba standardów i protokołów w zakresie stosowania SI. Praca podkreśla również potencjał zastosowania narzędzi wspieranych przez SI w monitorowaniu postępów choroby, co może przyczynić się do lepszych wyników leczenia oraz zwiększyć jakość życia pacjentów. W konkluzji, mimo wyzwań, perspektywy wykorzystania SI w diagnostyce czerniaka są obiecujące, a kontynuacja badań i rozwój technologiczny mogą znacznie przyspieszyć osiąganie postępów w tej krytycznej dziedzinie medycyny.
Bibliografia
Haenssle HA, Fink C, Schneiderbauer R, et al. Man against machine: diagnostic performance of a deep learning convolutional neural network for dermoscopic melanoma recognition in comparison to 58 dermatologists. Ann Oncol. 2018;29(8):1836-1842.
Swetter SM, Tsao H, Bichakjian CK, et al. Guidelines of care for the management of primary cutaneous melanoma. J Am Acad Dermatol. 2019;80(1):208-250.
Esteva A, Kuprel B, Novoa RA, et al. Dermatologist-level classification of skin cancer with deep neural networks. Nature. 2017;542(7639):115-118.
McCarthy J, Minsky ML, Rochester N, Shannon CE. A Proposal for the Dartmouth Summer Research Project on Artificial Intelligence. 1956.
Crevier D. AI: The Tumultuous History of the Search for Artificial Intelligence. Basic Books; 1993.
McCulloch WS, Pitts W. A logical calculus of the ideas immanent in nervous activity. The Bulletin of Mathematical Biophysics. 1943;5(4):115-133.
Samuel AL. Some Studies in Machine Learning Using the Game of Checkers. IBM J Res Dev. 1959;3(3):210-229.
LeCun Y, Bengio Y, Hinton G. Deep learning. Nature. 2015;521(7553):436-444.
Barnhill RL, Piepkorn M, Busam KJ. Pathology of Melanoma. W: Barnhill's Dermatopathology. 3rd ed. McGraw-Hill Education; 2014.
Davies H, Bignell GR, Cox C, et al. Mutations of the BRAF gene in human cancer. Nature. 2002;417(6892):949-954.
Quail DF, Joyce JA. Microenvironmental regulation of tumor progression and metastasis. Nat Med. 2013;19(11):1423-1437.
Flaherty KT, Hodi FS, Fisher DE. From genes to drugs: targeted strategies for melanoma. Nat Rev Cancer. 2012;12(5):349-361.
Scolyer RA, Thompson JF, Vilain RE. Amelanotic melanoma: a comprehensive review. Am J Surg Pathol. 2017;41(10):1387-1401.
Marghoob AA, Malvehy J, Braun RP. An Atlas of Dermoscopy. 2nd ed. CRC Press; 2012.
Elder DE, Massi D, Scolyer RA, Willemze R. WHO Classification of Skin Tumours. 4th ed. International Agency for Research on Cancer; 2018.
Scope A, Benvenuto-Andrade C, Agero AL, et al. In vivo reflectance confocal microscopy imaging of melanocytic skin lesions: consensus terminology glossary and illustrative images. J Am Acad Dermatol. 2007;57(4):644-658.
Menzies SW, Emery JD, Staples MP, et al. Impact of dermoscopic and histopathologic diagnostic information on the treatment decision in clinically equivocal melanocytic skin lesions. J Invest Dermatol. 2008;128(10):2388-2394.
Rajadhyaksha M, González S, Zavislan JM, et al. In vivo confocal scanning laser microscopy of human skin II: Advances in instrumentation and comparison with histology. J Invest Dermatol. 1999;113(3):293-303.
Manohar S, Vaartjes SE, van Hespen JC, et al. Initial results of in vivo non-invasive cancer imaging in the human breast using near-infrared photoacoustics. Opt Express. 2007;15(19):12277-12285.
Haenssle HA, Fink C, Schneiderbauer R, et al. Man against machine: diagnostic performance of a deep learning convolutional neural network for dermoscopic melanoma recognition in comparison to 58 dermatologists. Ann Oncol. 2018;29(8):1836-1842.
Esteva A, Kuprel B, Novoa RA, et al. Dermatologist-level classification of skin cancer with deep neural networks. Nature. 2017;542(7639):115-118.
Codella NCF, Gutman D, Celebi ME, et al. Skin lesion analysis toward melanoma detection: A challenge at the 2017 International Symposium on Biomedical Imaging (ISBI), hosted by the International Skin Imaging Collaboration (ISIC). IEEE Trans Med Imaging. 2018;37(1):272-282.
Combalia M, Codella NCF, Rotemberg V, et al. BCN20000: Dermoscopic Lesions in the Wild. Bioinformatics. 2019;35(21):4466-4472.
Tschandl P, Rosendahl C, Kittler H. The HAM10000 dataset: a large collection of multi-source dermatoscopic images of common pigmented skin lesions. Sci Data. 2018;5:180161.
Gostin LO, Halabi SF, Wilson K. Health Data and Privacy in the Digital Era. JAMA. 2018;320(3):233-234.
Codella NCF, Gutman D, Celebi ME, et al. Skin Lesion Analysis Toward Melanoma Detection: A Challenge at the 2017 International Symposium on Biomedical Imaging (ISBI), hosted by the International Skin Imaging Collaboration (ISIC). IEEE Trans Med Imaging. 2018;37(1):272-282.
Wolf JA, Moreau JF, Akilov O, et al. Diagnostic Inaccuracy of Smartphone Applications for Melanoma Detection. JAMA Dermatol. 2013;149(4):422-426.
Rajadhyaksha M, Marghoob A, Rossi A, et al. Reflectance confocal microscopy for skin diseases. Semin Cutan Med Surg. 2013;32(2):101-110.
Tognetti L, Cartocci A, Del Duca E, et al. Advanced digital health platforms in dermatology. Digital Health. 2020;6:1-10.
Haenssle HA, Fink C, Schneiderbauer R, et al. Man against machine: diagnostic performance of a deep learning convolutional neural network for dermoscopic melanoma recognition in comparison to 58 dermatologists. Ann Oncol. 2018;29(8):1836-1842.
Chartrand G, Cheng PM, Vorontsov E, et al. Deep learning: A primer for radiologists. Radiographics. 2017;37(7):2113-2131.
Topol EJ. High-performance medicine: the convergence of human and artificial intelligence. Nat Med. 2019;25(1):44-56.
Holzinger A, Biemann C, Pattichis CS, Kell DB. What do we need to build explainable AI systems for the medical domain? ArXiv Preprint ArXiv:1712.09923. 2017.
Cohen IG, Amarasingham R, Shah A, et al. The legal and ethical concerns that arise from using complex predictive analytics in health care. Health Aff. 2014;33(7):1139-1147.
Jha S, Topol EJ. Adapting to artificial intelligence: radiologists and pathologists as information specialists. JAMA. 2016;316(22):2353-2354.
Kelly CJ, Karthikesalingam A, Suleyman M, Corrado G, King D. Key challenges for delivering clinical impact with artificial intelligence. BMC Med. 2019;17(1):195.
Esteva A, Kuprel B, Novoa RA, et al. Dermatologist-level classification of skin cancer with deep neural networks. Nature. 2017;542(7639):115-118.
Liu Y, Kohlberger T, Norouzi M, et al. Artificial intelligence–based breast cancer nodal metastasis detection: Insights into the black box for pathologists. Arch Pathol Lab Med. 2019;143(7):859-868.
Rat C, Hild S, Rault Sérandour J, et al. Use of smartphone applications for melanoma detection in real life: a cross-sectional survey study. Dermatology. 2020;236(5):375-382.
Cohen IG, Amarasingham R, Shah A, et al. The legal and ethical concerns that arise from using complex predictive analytics in health care. Health Aff. 2014;33(7):1139-1147.
Topol EJ. High-performance medicine: the convergence of human and artificial intelligence. Nat Med. 2019;25(1):44-56.
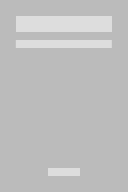
Zapowiedzi
Licencja

Utwór dostępny jest na licencji Creative Commons Uznanie autorstwa – Użycie niekomercyjne – Bez utworów zależnych 4.0 Międzynarodowe.