Innowacyjne Metody AI w Psychiatrii: Analiza Skuteczności i Perspektywy Rozwoju
Słowa kluczowe:
sztuczna inteligencja, uczenie maszynowe, głębokie uczenie, diagnostykaStreszczenie
Integracja Sztucznej Inteligencji (AI) w psychiatrii stanowi przełomowe posunięcie, oferując nowe możliwości m.in diagnozowania zaburzeń psychicznych. Niniejszy przegląd analizuje najnowszą literaturę w celu zbadania korzyści związanych z zastosowaniem technologii AI w psychiatrii. Autorzy przeprowadzili przegląd wykorzystując bazę danych PubMed, skupiając się na badaniach obejmujących AI, uczenie maszynowe i głębokie uczenie w kontekstach psychiatrycznych. Przegląd podkreśla znaczące postępy w diagnozowaniu i prognozowaniu problemów zdrowia psychicznego za pomocą AI. Techniki takie jak przetwarzanie języka naturalnego i głębokie uczenie przewyższają tradycyjne metody diagnostyczne, szczególnie w przewidywaniu psychozy i ryzyka samobójczego. Jednak wdrażanie AI w ustawieniach klinicznych napotyka na wyzwania, w tym jakość danych, potencjalne nadużycia oraz etyczne obawy. AI ma obiecujący potencjał, aby zwiększyć dokładność diagnoz psychiatrycznych i prognozować diagnostykę, leczenie oraz postępowanie chorób psychicznych. Jednak jego wdrożenie wymaga starannego rozważenia standardów etycznych i ciągłych badań interdyscyplinarnych w celu optymalizacji algorytmów dla zróżnicowanych warunków klinicznych. Przyszłe badania powinny również koncentrować się na rozwiązywaniu społecznych implikacji AI oraz utrzymywaniu dialogu między technologami, klinicystami, pacjentami i regulatorami, aby zapewnić odpowiedzialną integrację w psychiatrii.
Bibliografia
Bini SA. Artificial Intelligence, Machine Learning, Deep Learning, and Cognitive Computing: What Do These Terms Mean and How Will They Impact Health Care?. J Arthroplasty. 2018;33(8):2358-2361. doi:10.1016/j.arth.2018.02.067.
Ramesh AN, Kambhampati C, Monson JR, Drew PJ. Artificial intelligence in medicine. Ann R Coll Surg Engl. 2004;86(5):334-338. doi:10.1308/147870804290.
Balyen L, Peto T. Promising Artificial Intelligence-Machine Learning-Deep Learning Algorithms in Ophthalmology. Asia Pac J Ophthalmol (Phila). 2019;8(3):264-272. doi:10.22608/APO.2018479.
Hosny A, Parmar C, Quackenbush J, Schwartz LH, Aerts HJWL. Artificial intelligence in radiology. Nat Rev Cancer. 2018;18(8):500-510. doi:10.1038/s41568-018-0016-5.
Marneros A. Psychiatry's 200th birthday. Br J Psychiatry. 2008;193(1):1-3. doi:10.1192/bjp.bp.108.051367.
Fakhoury M. Artificial Intelligence in Psychiatry. Adv Exp Med Biol. 2019;1192:119-125. doi:10.1007/978-981-32-9721-0_6.
Dawson G, Bernier R. A quarter century of progress on the early detection and treatment of autism spectrum disorder. Dev Psychopathol. 2013;25(4 Pt 2):1455-1472. doi:10.1017/S0954579413000710.
Maenner MJ, Shaw KA, Baio J, et al. Prevalence of Autism Spectrum Disorder Among Children Aged 8 Years - Autism and Developmental Disabilities Monitoring Network, 11 Sites, United States, 2016. MMWR Surveill Summ. 2020;69(4):1-12. Published 2020 Mar 27. doi:10.15585/mmwr.ss6904a1.
Chekroud AM, Zotti RJ, Shehzad Z, et al. Cross-trial prediction of treatment outcome in depression: a machine learning approach. Lancet Psychiatry. 2016;3(3):243-250. doi:10.1016/S2215-0366(15)00471-X.
Bedi G, Carrillo F, Cecchi GA, et al. Automated analysis of free speech predicts psychosis onset in high-risk youths. NPJ Schizophr. 2015;1:15030. Published 2015 Aug 26. doi:10.1038/npjschz.2015.30.
Elvevåg B, Foltz PW, Rosenstein M, Delisi LE. An automated method to analyze language use in patients with schizophrenia and their first-degree relatives. J Neurolinguistics. 2010;23(3):270-284. doi:10.1016/j.jneuroling.2009.05.002.
Elvevåg B, Foltz PW, Weinberger DR, Goldberg TE. Quantifying incoherence in speech: an automated methodology and novel application to schizophrenia. Schizophr Res. 2007;93(1-3):304-316. doi:10.1016/j.schres.2007.03.001.
Kalmady SV, Greiner R, Agrawal R, et al. Towards artificial intelligence in mental health by improving schizophrenia prediction with multiple brain parcellation ensemble-learning. NPJ Schizophr. 2019;5(1):2. Published 2019 Jan 18. doi:10.1038/s41537-018-0070-8.
Dwyer D, Koutsouleris N. Annual Research Review: Translational machine learning for child and adolescent psychiatry. J Child Psychol Psychiatry. 2022;63(4):421-443. doi:10.1111/jcpp.13545.
Lin E, Lin CH, Lane HY. Precision Psychiatry Applications with Pharmacogenomics: Artificial Intelligence and Machine Learning Approaches. Int J Mol Sci. 2020;21(3):969. Published 2020 Feb 1. doi:10.3390/ijms21030969.
Värnik P. Suicide in the world. Int J Environ Res Public Health. 2012;9(3):760-771. doi:10.3390/ijerph9030760.
Pestian JP, Sorter M, Connolly B, et al. A Machine Learning Approach to Identifying the Thought Markers of Suicidal Subjects: A Prospective Multicenter Trial. Suicide Life Threat Behav. 2017;47(1):112-121. doi:10.1111/sltb.12312.
Walsh CG, Ribeiro JD, Franklin JC. Predicting suicide attempts in adolescents with longitudinal clinical data and machine learning. J Child Psychol Psychiatry. 2018;59(12):1261-1270. doi:10.1111/jcpp.12916.
Fernandes AC, Dutta R, Velupillai S, Sanyal J, Stewart R, Chandran D. Identifying Suicide Ideation and Suicidal Attempts in a Psychiatric Clinical Research Database using Natural Language Processing. Sci Rep. 2018;8(1):7426. Published 2018 May 9. doi:10.1038/s41598-018-25773-2.
Sanfelici R, Dwyer DB, Antonucci LA, Koutsouleris N. Individualized Diagnostic and Prognostic Models for Patients With Psychosis Risk Syndromes: A Meta-analytic View on the State of the Art. Biol Psychiatry. 2020;88(4):349-360. doi:10.1016/j.biopsych.2020.02.009.
Iniesta R, Malki K, Maier W, et al. Combining clinical variables to optimize prediction of antidepressant treatment outcomes. J Psychiatr Res. 2016;78:94-102. doi:10.1016/j.jpsychires.2016.03.016.
Nunes A, Ardau R, Berghöfer A, et al. Prediction of lithium response using clinical data. Acta Psychiatr Scand. 2020;141(2):131-141. doi:10.1111/acps.13122.
Eugene AR, Masiak J, Eugene B. Predicting lithium treatment response in bipolar patients using gender-specific gene expression biomarkers and machine learning. F1000Res. 2018;7:474. Published 2018 Apr 18. doi:10.12688/f1000research.14451.3.
Ortiz A, Munilla J, Górriz JM, Ramírez J. Ensembles of Deep Learning Architectures for the Early Diagnosis of the Alzheimer's Disease. Int J Neural Syst. 2016;26(7):1650025. doi:10.1142/S0129065716500258.
Nayak CS, Anilkumar AC. EEG Normal Waveforms. In: StatPearls. Treasure Island (FL): StatPearls Publishing; July 24, 2023.
St. Louis EK, Frey LC, Britton JW, Frey LC, Hopp JL, et al., eds. Electroencephalography (EEG): An Introductory Text and Atlas of Normal and Abnormal Findings in Adults, Children, and Infants. Chicago: American Epilepsy Society; 2016.
Watts D, Pulice RF, Reilly J, Brunoni AR, Kapczinski F, Passos IC. Predicting treatment response using EEG in major depressive disorder: A machine-learning meta-analysis. Transl Psychiatry. 2022;12(1):332. Published 2022 Aug 12. doi:10.1038/s41398-022-02064-z.
de Bardeci M, Ip CT, Olbrich S. Deep learning applied to electroencephalogram data in mental disorders: A systematic review. Biol Psychol. 2021;162:108117. doi:10.1016/j.biopsycho.2021.108117.
Terra, M., Baklola, M., Ali, S. et al. Opportunities, applications, challenges and ethical implications of artificial intelligence in psychiatry: a narrative review. Egypt J Neurol Psychiatry Neurosurg 59, 80 (2023). doi:10.1186/s41983-023-00681-z.
Tortora L. Beyond Discrimination: Generative AI Applications and Ethical Challenges in Forensic Psychiatry. Front Psychiatry. 2024;15:1346059. Published 2024 Mar 8. doi:10.3389/fpsyt.2024.1346059.
Monteith S, Glenn T, Geddes J, Whybrow PC, Achtyes E, Bauer M. Expectations for Artificial Intelligence (AI) in Psychiatry. Curr Psychiatry Rep. 2022;24(11):709-721. doi:10.1007/s11920-022-01378-5.
Loch AA, Lopes-Rocha AC, Ara A, et al. Ethical Implications of the Use of Language Analysis Technologies for the Diagnosis and Prediction of Psychiatric Disorders. JMIR Ment Health. 2022;9(11):e41014. Published 2022 Nov 1. doi:10.2196/41014.
Wiese W, Friston KJ. AI ethics in computational psychiatry: From the neuroscience of consciousness to the ethics of consciousness. Behav Brain Res. 2022;420:113704. doi:10.1016/j.bbr.2021.113704.
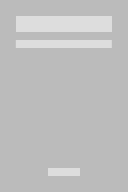
Opublikowane
Licencja

Utwór dostępny jest na licencji Creative Commons Uznanie autorstwa – Użycie niekomercyjne – Bez utworów zależnych 4.0 Międzynarodowe.