Etyka wykorzystania sztucznej inteligencji w opiece zdrowotnej
Słowa kluczowe:
sztuczna inteligencjaStreszczenie
Ingerencja sztucznej inteligencji (AI) rewolucjonizuje obszar opieki zdrowotnej, zwiastując nową erę innowacji medycznych, których celem jest usprawnienie procesów diagnostycznych i lepsza opieka nad pacjentami. Niemniej jednak, równolegle z jej rozwojem, uwidocznieniu ulega szereg implikacji etycznych związanych z prywatnością, uczciwością, bezpieczeństwem, przejrzystością i odpowiedzialnością za narzędzia AI, proces ich wdrażania i zastosowania. Jedynym sposobem na wykorzystanie potencjału, jaki niosą ze sobą narzędzi AI, jest stosowanie podstawowych zasad etyki oraz poszanowanie praw pacjenta. Celem poniższego rozdziału jest przybliżenie kwestii etycznych wykorzystania AI, istniejących ram prawnych dotyczących jej stosowania oraz ukazanie obawy, które trapią głównych potencjalnych beneficjentów procesu - pacjentów.
Bibliografia
Saheb T, Saheb T, Carpenter DO. Mapping research strands of ethics of artificial intelligence in healthcare: A bibliometric and content analysis. Computers in Biology and Medicine. 2021;135:104660. doi:10.1016/j.compbiomed.2021.104660
Rajpurkar P, Chen E, Banerjee O, Topol EJ. AI in health and medicine. Nat Med. 2022;28(1):31-38. doi:10.1038/s41591-021-01614-0
Airenti G. The Cognitive Bases of Anthropomorphism: From Relatedness to Empathy. Int J of Soc Robotics. 2015;7(1):117-127. doi:10.1007/s12369-014-0263-x)
Epley N, Waytz A, Cacioppo JT. On seeing human: A three-factor theory of anthropomorphism. Psychological Review. 2007;114(4):864-886. doi:10.1037/0033-295x.114.4.864
Epley N, Waytz A, Akalis S, Cacioppo JT. When We Need A Human: Motivational Determinants of Anthropomorphism. Social Cognition. 2008;26(2):143-155. doi:10.1521/soco.2008.26.2.143
Epley N. A Mind like Mine: The Exceptionally Ordinary Underpinnings of Anthropomorphism. Journal of the Association for Consumer Research. 2018;3(4):591-598. doi:10.1086/699516
Bartneck C. Robots In The Theatre And The Media. Unpublished. Published online 2013. doi:10.13140/RG.2.2.28798.79682
Hartzog, W. Unfair and deceptive robots. Maryland Law Review. 2015. 785: 74
Kaminski, M., M. Rueben, C. Grimm, and W. D. Smart. 2017. Averting robot eyes. Maryland Law Review 76:983
Lyons JB, Hobbs K, Rogers S, Clouse SH. Responsible (use of) AI. Front Neuroergonomics. 2023;4. doi:10.3389/fnrgo.2023.1201777
Fernández-Alemán JL, Señor IC, Lozoya PÁO, Toval A. Security and privacy in electronic health records: A systematic literature review. Journal of Biomedical Informatics. 2013;46(3):541-562. doi:10.1016/j.jbi.2012.12.003
Obermeyer Z, Powers B, Vogeli C, Mullainathan S. Dissecting racial bias in an algorithm used to manage the health of populations. Science. 2019;366(6464):447-453. doi:10.1126/science.aax2342
Mittelstadt BD, Allo P, Taddeo M, Wachter S, Floridi L. The ethics of algorithms: Mapping the debate. Big Data & Society. 2016;3(2):205395171667967. doi:10.1177/2053951716679679
Calo R, Froomkin AM, Kerr I. Artificial intelligence policy: a primer and roadmap. SSRN Electron J. 2017;51:399
Darby A, Strum MW, Holmes E, Gatwood J. A Review of Nutritional Tracking Mobile Applications for Diabetes Patient Use. Diabetes Technology & Therapeutics. 2016;18(3):200-212. doi:10.1089/dia.2015.0299
Price WN II, Cohen IG. Privacy in the age of medical big data. Nat Med. 2019;25(1):37-43. doi:10.1038/s41591-018-0272-7
Suresh H, Guttag JV. A Framework for Understanding Sources of Harm throughout the Machine Learning Life Cycle. arXiv. Published online 2019. doi:10.48550/ARXIV.1901.10002
Calders T, Verwer S. Three naive Bayes approaches for discrimination-free classification. Data Min Knowl Disc. 2010;21(2):277-292. doi:10.1007/s10618-010-0190-x
Dwork C, Hardt M, Pitassi T, Reingold O, Zemel R. Fairness Through Awareness. Published online 2011. doi:10.48550/ARXIV.1104.3913
Sabry F, Eltaras T, Labda W, Alzoubi K, Malluhi Q. Machine Learning for Healthcare Wearable Devices: The Big Picture. Wu Y, ed. Journal of Healthcare Engineering. 2022;2022:1-25. doi:10.1155/2022/4653923
European Data Protection Board. Guidelines 3/2019 on processing of personal data through video devices. 2019
Khoury MJ, Bowen S, Dotson WD, et al. Health equity in the implementation of genomics and precision medicine: A public health imperative. Genetics in Medicine. 2022;24(8):1630-1639. doi:10.1016/j.gim.2022.04.009
Istasy P, Lee WS, Iansavichene A, et al. The Impact of Artificial Intelligence on Health Equity in Oncology: Scoping Review. J Med Internet Res. 2022;24(11):e39748. doi:10.2196/39748
Corbett-Davies S, Gaebler JD, Nilforoshan H, Shroff R, Goel S. The Measure and Mismeasure of Fairness. arXiv. Published online 2018. doi:10.48550/ARXIV.1808.00023
Buolamwini J, Gebru T. Gender shades: Intersectional accuracy disparities in commercial gender classification. Proceedings of the 1st Conference on Fairness, Accountability and Transparency. 2018:77–91
Wachter S, Mittelstadt B, Floridi L. Transparent, explainable, and accountable AI for robotics. Sci Robot. 2017;2(6). doi:10.1126/scirobotics.aan6080
Coeckelbergh M. Artificial Intelligence, Responsibility Attribution, and a Relational Justification of Explainability. Sci Eng Ethics. 2019;26(4):2051-2068. doi:10.1007/s11948-019-00146-8
Caruana R, Lou Y, Gehrke J, Koch P, Sturm M, Elhadad N. Intelligible Models for HealthCare. Proceedings of the 21th ACM SIGKDD International Conference on Knowledge Discovery and Data Mining. Published online August 10, 2015. doi:10.1145/2783258.2788613
Ribeiro MT, Singh S, Guestrin C. “Why Should I Trust You?” Proceedings of the 22nd ACM SIGKDD International Conference on Knowledge Discovery and Data Mining. Published online August 13, 2016. doi:10.1145/2939672.2939778
Obasa AE, Palk AC. Responsible application of artificial intelligence in health care. S Afr J Sci. 2023;119(5/6). doi:10.17159/sajs.2023/14889
Hagos DH, Rawat DB. Recent Advances in Artificial Intelligence and Tactical Autonomy: Current Status, Challenges, and Perspectives. Sensors. 2022;22(24):9916. doi:10.3390/s22249916
Amann J, Blasimme A, Vayena E, Frey D, Madai VI. Explainability for artificial intelligence in healthcare: a multidisciplinary perspective. BMC Med Inform Decis Mak. 2020;20(1).
Fehr J, Jaramillo-Gutierrez G, Oala L, et al. Piloting a Survey-Based Assessment of Transparency and Trustworthiness with Three Medical AI Tools. Healthcare. 2022;10(10):1923. doi:10.3390/healthcare10101923 doi:10.1186/s12911-020-01332-6
Bjerring JC, Busch J. Artificial Intelligence and Patient-Centered Decision-Making. Philos Technol. 2020;34(2):349-371. doi:10.1007/s13347-019-00391-6
Chaddad A, Peng J, Xu J, Bouridane A. Survey of Explainable AI Techniques in Healthcare. Sensors. 2023;23(2):634. doi:10.3390/s23020634
Politi MC, Dizon DS, Frosch DL, Kuzemchak MD, Stiggelbout AM. Importance of clarifying patients’ desired role in shared decision making to match their level of engagement with their preferences. BMJ. 2013;347(dec02 1):f7066-f7066. doi:10.1136/bmj.f7066
Stacey D, Légaré F, Lewis K, et al. Decision aids for people facing health treatment or screening decisions. Cochrane Database of Systematic Reviews. 2017;2017(4). doi:10.1002/14651858.cd001431.pub5
Amann J, Blasimme A, Vayena E, Frey D, Madai VI. Explainability for artificial intelligence in healthcare: a multidisciplinary perspective. BMC Med Inform Decis Mak. 2020;20(1). doi:10.1186/s12911-020-01332-6
Elwyn G, Frosch D, Thomson R, et al. Shared Decision Making: A Model for Clinical Practice. J GEN INTERN MED. 2012;27(10):1361-1367. doi:10.1007/s11606-012-2077-6
Sinsky C, Colligan L, Li L, et al. Allocation of Physician Time in Ambulatory Practice: A Time and Motion Study in 4 Specialties. Ann Intern Med. 2016;165(11):753. doi:10.7326/m16-0961
Emanuel EJ, Wachter RM. Artificial Intelligence in Health Care. JAMA. 2019;321(23):2281. doi:10.1001/jama.2019.4914
Ayala Solares JR, Diletta Raimondi FE, Zhu Y, et al. Deep learning for electronic health records: A comparative review of multiple deep neural architectures. Journal of Biomedical Informatics. 2020;101:103337. doi:10.1016/j.jbi.2019.103337
World Medical Association Declaration of Helsinki. JAMA. 2013;310(20):2191. doi:10.1001/jama.2013.281053
van Kolfschooten H, Shachar C. The Council of Europe’s AI Convention (2023–2024): Promises and pitfalls for health protection. Health Policy. 2023;138:104935. doi:10.1016/j.healthpol.2023.104935
World Health Organisation. Digital health. 2019
Summary of recommendations regarding COVID ‐19 in children with diabetes: Keep Calm and Mind your Diabetes Care and Public Health Advice. Pediatr Diabetes. 2020;21(3):413-414. doi:10.1111/pedi.13013
Food and Drug Administration. Proposed regulatory framework for modifications to artificial intelligence/machine learning (AI/ML)-based software as a medical device (SaMD). 2019
Naik N, Hameed BMZ, Shetty DK, et al. Legal and Ethical Consideration in Artificial Intelligence in Healthcare: Who Takes Responsibility? Front Surg. 2022;9. doi:10.3389/fsurg.2022.862322
Brady AP, Neri E. Artificial Intelligence in Radiology—Ethical Considerations. Diagnostics. 2020;10(4):231. doi:10.3390/diagnostics10040231
IEEE Global Initiative on Ethics of Autonomous and Intelligent Systems. Ethically aligned design: a vision for prioritizing human well-being with autonomous and intelligent systems. 2019
Nagai H, Nakazawa E, Akabayashi A. The creation of the Belmont Report and its effect on ethical principles: a historical study. Monash Bioeth Rev. 2022;40(2):157-170. doi:10.1007/s40592-022-00165-5
Winfield AFT, Booth S, Dennis LA, et al. IEEE P7001: A Proposed Standard on Transparency. Front Robot AI. 2021;8. doi:10.3389/frobt.2021.665729
Ruger JP. Ethics of the social determinants of health. The Lancet. 2004;364(9439):1092-1097. doi:10.1016/s0140-6736(04)17067-0
Sivarajah U, Wang Y, Olya H, Mathew S. Responsible Artificial Intelligence (AI) for Digital Health and Medical Analytics. Inf Syst Front. 2023;25(6):2117-2122. doi:10.1007/s10796-023-10412-7
Esmaeilzadeh P, Mirzaei T, Dharanikota S. Patients’ Perceptions Toward Human–Artificial Intelligence Interaction in Health Care: Experimental Study. J Med Internet Res. 2021;23(11):e25856. doi:10.2196/25856
Xu J. Overtrust of Robots in High-Risk Scenarios. Proceedings of the 2018 AAAI/ACM Conference on AI, Ethics, and Society. Published online December 27, 2018. doi:10.1145/3278721.3278786
Reddy S, Allan S, Coghlan S, Cooper P. A governance model for the application of AI in health care. Journal of the American Medical Informatics Association. 2019;27(3):491-497. doi:10.1093/jamia/ocz192
Vayena E, Blasimme A, Cohen IG. Machine learning in medicine: Addressing ethical challenges. PLoS Med. 2018;15(11):e1002689. doi:10.1371/journal.pmed.1002689
Lu L, Cai R, Gursoy D. Developing and validating a service robot integration willingness scale. International Journal of Hospitality Management. 2019;80:36-51. doi:10.1016/j.ijhm.2019.01.005
Vayena E, Blasimme A, Cohen IG. Machine learning in medicine: Addressing ethical challenges. PLoS Med. 2018;15(11):e1002689. doi:10.1371/journal.pmed.1002689
Gursoy D, Chi OH, Lu L, Nunkoo R. Consumers acceptance of artificially intelligent (AI) device use in service delivery. International Journal of Information Management. 2019;49:157-169. doi:10.1016/j.ijinfomgt.2019.03.008
Hair JF, Ringle CM, Sarstedt M. PLS-SEM: Indeed a Silver Bullet. Journal of Marketing Theory and Practice. 2011;19(2):139-152. doi:10.2753/mtp1069-6679190202
Cath C. Governing artificial intelligence: ethical, legal and technical opportunities and challenges. Phil Trans R Soc A. 2018;376(2133):20180080. doi:10.1098/rsta.2018.0080
Reddy S, Allan S, Coghlan S, Cooper P. A governance model for the application of AI in health care. Journal of the American Medical Informatics Association. 2019;27(3):491-497. doi:10.1093/jamia/ocz192
Beets B, Newman TP, Howell EL, Bao L, Yang S. Surveying Public Perceptions of Artificial Intelligence in Health Care in the United States: Systematic Review. J Med Internet Res. 2023;25:e40337. doi:10.2196/40337
https://www.monmouth.edu/polling-institute/reports/monmouthpoll_us_042015/
https://www.icpsr.umich.edu/web/ICPSR/studies/34601/versions/V1
Yap A, Wilkinson B, Chen E, et al. Patients Perceptions of Artificial Intelligence in Diabetic Eye Screening. Asia-Pacific Journal of Ophthalmology. 2022;11(3):287-293. doi:10.1097/apo.0000000000000525
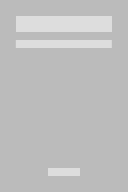
Zapowiedzi
Licencja

Utwór dostępny jest na licencji Creative Commons Uznanie autorstwa – Użycie niekomercyjne – Bez utworów zależnych 4.0 Międzynarodowe.