Ocena wieku kostnego na podstawie rentgenowskiego obrazu ręki: różnice w ocenie między lekarzem a sztuczną inteligencją: przegląd systematyczny
Słowa kluczowe:
uczenie maszynowe, wiek kostny, RTG, dłoń, automatyczna ocena, metaanalizaStreszczenie
Ocena wieku kostnego odgrywa kluczową rolę w diagnostyce i monitorowaniu schorzeń endokrynologicznych i ortopedycznych u dzieci. Chociaż tradycyjna, ręczna ocena przeprowadzana przez lekarzy od dawna jest uważana za złoty standard, najnowsze postępy technologiczne wprowadzają systemy sztucznej inteligencji (AI) jako potencjalną alternatywę. Te systemy sztucznej inteligencji są obiecujące, jeśli chodzi o osiąganie wyników porównywalnych z oceną ręczną, ale przynoszą dodatkowe korzyści, takie jak obiektywność, wysoka wydajność i mniejsze obciążenie lekarzy. W artykule dokonano przeglądu literatury na temat systematycznego porównywania ocen wieku kostnego przeprowadzanych przez lekarzy z algorytmami AI wykorzystującymi techniki uczenia maszynowego. Metodologia zakłada systematyczne przeszukiwanie bazy PubMed, w wyniku której uzyskano 16 odpowiednich wyników, które koncentrują się na automatycznej ocenie wieku przy użyciu sztucznej inteligencji w porównaniu z metodami tradycyjnymi. Wybrane badania wskazują na postęp i potencjał w ocenie wieku kostnego w oparciu o sztuczną inteligencję, wykazując poprawę dokładności i wydajności. Wyzwania obejmują jednak ograniczoną zdolność sztucznej inteligencji do wykrywania złożonych nieprawidłowości kości lub uwzględniania kontekstu klinicznego, co podkreśla potrzebę dalszych badań w celu zrozumienia pełnego zakresu i ograniczeń sztucznej inteligencji w tej dziedzinie. Ogólnie rzecz biorąc, choć ocena wieku kostnego wspomagana sztuczną inteligencją wykazuje porównywalną dokładność i skuteczność z oceną lekarza, należy ją postrzegać raczej jako narzędzie uzupełniające niż całkowite zamiennik, przy czym do jej niezawodnego i etycznego wdrożenia potrzebne są ciągłe badania i walidacja.
Bibliografia
Monill‐González A, Rovira‐Calatayud L, d’Oliveira NG, Ustrell‐Torrent JM. Artificial intelligence in orthodontics: Where are we now? A scoping review. Orthodontics & Craniofacial Research. 2021;24(S2):6-15. doi:https://doi.org/10.1111/ocr.12517
Winnah Wu-in Lea, Suk Joo Hong, Nam HK, Kang W, Yang Z, Noh EJ. External validation of deep learning-based bone-age software: a preliminary study with real world data. Scientific Reports. 2022;12(1). doi:https://doi.org/10.1038/s41598-022-05282-z
Lee BD, Lee MS. Automated Bone Age Assessment Using Artificial Intelligence: The Future of Bone Age Assessment. Korean Journal of Radiology. 2021;22. doi:https://doi.org/10.3348/kjr.2020.0941
Booz C, Yel I, Wichmann JL, et al. Artificial intelligence in bone age assessment: accuracy and efficiency of a novel fully automated algorithm compared to the Greulich-Pyle method. European Radiology Experimental. 2020;4(1). doi:https://doi.org/10.1186/s41747-019-0139-9
Zhou XL, Wang EG, Lin Q, et al. Diagnostic performance of convolutional neural network-based Tanner-Whitehouse 3 bone age assessment system. Quantitative Imaging in Medicine and Surgery. 2020;10(3):657-667. doi:https://doi.org/10.21037/qims.2020.02.20
Huang S, Su Z, Liu S, et al. Combined assisted bone age assessment and adult height prediction methods in Chinese girls with early puberty: analysis of three artificial intelligence systems. Pediatric Radiology. 2022;53(6):1108-1116. doi:https://doi.org/10.1007/s00247-022-05569-3
Cho DH, Yun Sun Choi, Oh H, Young Min Ahn, Seo JY. Accuracy of predicted adult height using the Greulich-Pyle method and artificial intelligence medical device. Clinical and experimental pediatrics (Online). 2023;66(3):145-147. doi:https://doi.org/10.3345/cep.2022.01116
Xi W, Zhou B, Gong P, et al. Artificial Intelligence–Assisted Bone Age Assessment to Improve the Accuracy and Consistency of Physicians With Different Levels of Experience. Frontiers in Pediatrics. 2022;10. doi:https://doi.org/10.3389/fped.2022.818061
Lee KC, Lee KH, Kang CH, et al. Clinical Validation of a Deep Learning-Based Hybrid (Greulich-Pyle and Modified Tanner-Whitehouse) Method for Bone Age Assessment. Korean Journal of Radiology. 2021;22(12):2017. doi:https://doi.org/10.3348/kjr.2020.1468
Spampinato C, Palazzo S, Giordano D, Aldinucci M, Leonardi R. Deep learning for automated skeletal bone age assessment in X-ray images. Medical Image Analysis. 2017;36:41-51. doi:https://doi.org/10.1016/j.media.2016.10.010
Lee BD, Lee MS. Automated Bone Age Assessment Using Artificial Intelligence: The Future of Bone Age Assessment. Korean Journal of Radiology. 2021;22. doi:https://doi.org/10.3348/kjr.2020.0941
Booz C, Yel I, Wichmann JL, et al. Artificial intelligence in bone age assessment: accuracy and efficiency of a novel fully automated algorithm compared to the Greulich-Pyle method. European Radiology Experimental. 2020;4(1). doi:https://doi.org/10.1186/s41747-019-0139-9
Zhou ZH. Machine Learning. Springer Nature; 2021. Accessed December 16, 2023. https://books.google.com/books?hl=pl&lr=&id=ctM-EAAAQBAJ&oi=fnd&pg=PR6&dq=machine+learning+&ots=o_Ij_5Ts3p&sig=2JaTsIT6HV1k4dDLie-0JGwuPB8
Hirschmann A, Cyriac J, Stieltjes B, Kober T, Richiardi J, Omoumi P. Artificial Intelligence in Musculoskeletal Imaging: Review of Current Literature, Challenges, and Trends. Seminars in Musculoskeletal Radiology. 2019;23(03):304-311. doi:https://doi.org/10.1055/s-0039-1684024
Han XG, Tian W. Artificial intelligence in orthopedic surgery: current state and future perspective. Chinese Medical Journal. 2019;132(21):2521-2523. doi:https://doi.org/10.1097/CM9.0000000000000479
Zhao K, Ma S, Sun Z, et al. Effect of AI-assisted software on inter- and intra-observer variability for preschool children X-ray bone age assessment. Research Square (Research Square). Published online June 17, 2022. doi:https://doi.org/10.21203/rs.3.rs-1737407/v1
Pan X, Zhao Y, Chen H, Wei D, Zhao C, Wei Z. Fully Automated Bone Age Assessment on Large-Scale Hand X-Ray Dataset. International Journal of Biomedical Imaging. 2020;2020:1-12. doi:https://doi.org/10.1155/2020/8460493
Al SKC et. Novel approach to determine age and gender from wrist x-ray images. Turkish Journal of Computer and Mathematics Education (TURCOMAT). 2021;12(3):5215-5229. Accessed December 16, 2023. https://www.turcomat.org/index.php/turkbilmat/article/view/2151
Kim SU, Oh S, Lee KH, Chang Ho Kang, Kyung Sik Ahn. Improvement of Bone Age Assessment Using a Deep Learning Model in Young Children: Significance of Carpal Bone Analysis. Iranian Journal of Radiology. 2023;20(2). doi:https://doi.org/10.5812/iranjradiol-136311
Simu S, Lal S, Nagarsekar P, Naik A. Fully automatic ROI extraction and edge-based segmentation of radius and ulna bones from hand radiographs. Biocybernetics and Biomedical Engineering. 2017;37(4):718-732. doi:https://doi.org/10.1016/j.bbe.2017.07.004
Liu R, Jia Y, He X, et al. Ensemble Learning with Multiclassifiers on Pediatric Hand Radiograph Segmentation for Bone Age Assessment. Chen JC, ed. International Journal of Biomedical Imaging. 2020;2020:1-12. doi:https://doi.org/10.1155/2020/8866700
Effect of AI-assisted software on inter- and intra-observer variability for preschool children X-ray bone age assessment. www.researchsquare.com. Published July 12, 2022. Accessed December 16, 2023. https://www.researchsquare.com/article/rs-1737407/v2
Rajitha B, Agarwal S. Segmentation of Epiphysis Region-of-Interest (EROI) using texture analysis and clustering method for hand bone age assessment. Multimedia Tools and Applications. 2021;81(1):1029-1054. doi:https://doi.org/10.1007/s11042-021-11531-6
Deng Y, Chen Yonglu, He Q, et al. Bone age assessment from articular surface and epiphysis using deep neural networks. Mathematical Biosciences and Engineering. 2023;20(7):13133-13148. doi:https://doi.org/10.3934/mbe.2023585
Adeshina S, Cootes T, Adams J. Assessment of Different Structures for Predicting Bone Age using Statistical Appearance Models. INTERNATIONAL JOURNAL OF COMPUTER TECHNIQUES. 2017;4(5). Accessed December 16, 2023. http://www.ijctjournal.org/Volume4/Issue5/IJCT-V4I5P10.pdf
Witsarut Upalananda, Kittichai Wantanajittikul, Sakarat Na Lampang, Apirum Janhom. Semi-automated technique to assess the developmental stage of mandibular third molars for age estimation. Australian Journal of Forensic Sciences. 2021;55(1):23-33. doi:https://doi.org/10.1080/00450618.2021.1882570
Welter P, Deserno TM, Fischer B, Günther RW, Spreckelsen C. Towards case-based medical learning in radiological decision making using content-based image retrieval. BMC Medical Informatics and Decision Making. 2011;11(1). doi:https://doi.org/10.1186/1472-6947-11-68
Wu H, Ye X, Jiang Y, et al. A comparative study of multiple deep learning models based on multi-input resolution for breast ultrasound images. Frontiers. May 23, 2022. Accessed December 17, 2023. https://www.frontiersin.org/journals/oncology/articles/10.3389/fonc.2022.869421/full.
Li Z, Chen W, Ju Y, et al. Bone age assessment based on deep neural networks with annotation-free cascaded critical bone region extraction. Frontiers. February 6, 2023. Accessed December 17, 2023. https://www.frontiersin.org/articles/10.3389/frai.2023.1142895/full.
Wang X, Zhou B, Gong P, et al. Artificial Intelligence–Assisted Bone age assessment to improve the accuracy and consistency of physicians with different levels of experience. Frontiers. January 26, 2022. Accessed December 17, 2023. https://www.frontiersin.org/articles/10.3389/fped.2022.818061/full.
Nang KM, Ismail AJ, Tangaperumal A, et al. Forensic age estimation in living children: How accurate is the greulich-pyle method in Sabah, East Malaysia? Frontiers. May 18, 2023. Accessed December 17, 2023. https://www.frontiersin.org/articles/10.3389/fped.2023.1137960/full.
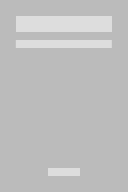
Opublikowane
Licencja

Utwór dostępny jest na licencji Creative Commons Uznanie autorstwa – Użycie niekomercyjne – Bez utworów zależnych 4.0 Międzynarodowe.