Wykorzystanie sztucznej inteligencji w analizie obrazów endoskopowych: Automatyczna detekcja zmian patologicznych
Słowa kluczowe:
sztuczna inteligencja, AI, endoskopia, gastroenterologia, sieci neuronoweStreszczenie
Sztuczna inteligencja (SI) stanowi obiecującą perspektywę dla dziedziny medycznej diagnostyki poprzez jej integrację w analizę endoskopową, co przynosi znaczące rezultaty w automatycznym wykrywaniu zmian patologicznych. W ostatnich latach, wykorzystanie konwolucyjnych sieci neuronowych w analizie obrazów endoskopowych zdobyło znaczną uwagę, co zaowocowało doskonałością w detekcji oraz charakteryzacji zmian patologicznych. Zautomatyzowane systemy do analizy obrazów endoskopowych nie tylko wykazały obiecujące wyniki, lecz także posiadają potencjał rewolucyjnego wpływu na procesy diagnostyczne, przyczyniając się do poprawy precyzji i efektywności diagnostycznej. Wdrożenie sztucznej inteligencji w interpretacji obrazów medycznych znacząco przyspieszyło postęp w dziedzinie endoskopii, wykazując nadzwyczajny potencjał do dalszych usprawnień w diagnostyce medycznej. Zastosowanie SI w analizie obrazów endoskopowych otwiera nowe możliwości w diagnostyce medycznej, umożliwiając szybsze i bardziej precyzyjne wykrywanie zmian patologicznych, co może przyczynić się do poprawy wyników pacjentów oraz efektywności opieki zdrowotnej. Ponadto, integracja SI w endoskopii może przyczynić się do standaryzacji analizy między różnymi endoskopistami, redukując zmienność między obserwatorami oraz umożliwiając bardziej efektywne wykorzystanie zasobów endoskopowych. Dodatkowo, SI może pomóc w analizie dynamicznych zmian na obrazach endoskopowych, takich jak rozpoznawanie wzorców naczyniowych lub błon śluzowych, co może być wskaźnikiem postępu choroby lub reakcji na leczenie. Wnioski z dotychczasowych badań wskazują na obiecujący potencjał SI w rewolucjonizowaniu dziedziny analizy endoskopowej i poprawianiu wyników pacjentów. Dalsze badania i rozwój algorytmów SI w analizie endoskopowej mogą przynieść dalsze korzyści, takie jak zwiększenie dokładności diagnostycznej, poprawa efektywności oraz udzielanie wskazówek w czasie rzeczywistym podczas procedur endoskopowych.
Bibliografia
Lee, B D., & Lee, M S. (2021, January 1). Automated Bone Age Assessment Using Artificial Intelligence: The Future of Bone Age Assessment. https://doi.org/10.3348/kjr.2020.0941
Sullivan P, Gupta S, Powers PD, Marya NB. Artificial Intelligence Research and Development for Application in Video Capsule Endoscopy. Gastrointestinal Endoscopy Clinics of North America. 2021;31(2):387-397. doi:https://doi.org/10.1016/j.giec.2020.12.009
Shen D, Wu G, Suk HI. Deep Learning in Medical Image Analysis. Annual Review of Biomedical Engineering. 2017;19(1):221-248. doi:https://doi.org/10.1146/annurev-bioeng-071516-044442
Gan T, Liu S, Yang J, Zeng B, Yang L. A pilot trial of Convolution Neural Network for automatic retention-monitoring of capsule endoscopes in the stomach and duodenal bulb. Scientific Reports. 2020;10(1):4103. doi:https://doi.org/10.1038/s41598-020-60969-5
Li L, Chen Y, Shen Z, et al. Convolutional neural network for the diagnosis of early gastric cancer based on magnifying narrow band imaging. Gastric Cancer. 2019;23(1):126-132. doi:https://doi.org/10.1007/s10120-019-00992-2
Review on the Applications of Deep Learning in the Analysis of Gastrointestinal Endoscopy Images | IEEE Journals & Magazine | IEEE Xplore. ieeexplore.ieee.org. Accessed December 28, 2023. https://ieeexplore.ieee.org/document/8853349/
Song Y, Mao X, Zhou X, et al. Use of Artificial Intelligence to Improve the Quality Control of Gastrointestinal Endoscopy. Frontiers in Medicine. 2021;8. doi:https://doi.org/10.3389/fmed.2021.709347
Fusco, R., Piccirillo, A., Sansone, M., Granata, V., Rubulotta, M R., Petrosino, T., Barretta, M L., Vallone, P., Giacomo, R D., Esposito, E., Bonito, M D., & Petrillo, A. (2021, April 30). Radiomics and Artificial Intelligence Analysis with Textural Metrics Extracted by Contrast-Enhanced Mammography in the Breast Lesions Classification. https://doi.org/10.3390/diagnostics11050815
Goel, C., Kumar, A., Dubey, S., & Srivastava, V. (2020, August 17). Efficient Deep Network Architecture for COVID-19 Detection Using Computed Tomography Images. https://doi.org/10.1101/2020.08.14.20170290
Yu, X., Song, Z., Zou, S., You, Y., Cui, J., Wang, S., Ku, C., Wu, X., Xue, X., Han, W., & Zhou, W. (2022, June 9). Artificial Intelligence Assisted Topographic Mapping System for Endoscopic Submucosal Dissection Specimens.
Fusco R, Grassi R, Granata V, et al. Artificial Intelligence and COVID-19 Using Chest CT Scan and Chest X-ray Images: Machine Learning and Deep Learning Approaches for Diagnosis and Treatment. Journal of Personalized Medicine. 2021;11(10):993. doi:https://doi.org/10.3390/jpm11100993
Goel N, Kaur S, Gunjan D, Mahapatra SJ. Dilated CNN for abnormality detection in wireless capsule endoscopy images. Soft Computing. 2022;26(3):1231-1247. doi:https://doi.org/10.1007/s00500-021-06546-y
Yu, X., Song, Z., Zou, S., You, Y., Cui, J., Wang, S., Ku, C., Wu, X., Xue, X., Han, W., & Zhou, W. (2022, June 9). Artificial Intelligence Assisted Topographic Mapping System for Endoscopic Submucosal Dissection Specimens.
Ribeiro E, Uhl A, Wimmer G, Häfner M. Transfer Learning for Colonic Polyp Classification Using Off-the-Shelf CNN Features. Lecture Notes in Computer Science. Published online January 1, 2017:1-13. doi:https://doi.org/10.1007/978-3-319-54057-3_1
Gaeed AK, Khalida Alwan Mansour. Effectiveness of the Instructional Program on Physical and Psychological Status of Patients Undergoing Upper Gastrointestinal Endoscopy at Baghdad Teaching Hospitals. kufa Journal for Nursing sciences. 2015;5(1):137-147. doi:https://doi.org/10.36321/kjns.vi20151.3160
Škiljić S, Budrovac D, Cicvarić A, Nenad Nešković, Slavica Kvolik. Advances in Analgosedation and Periprocedural Care for Gastrointestinal Endoscopy. Life. 2023;13(2):473-473. doi:https://doi.org/10.3390/life13020473
Shin K, Lee JS, Lee JY, et al. An Image Turing Test on Realistic Gastroscopy Images Generated by Using the Progressive Growing of Generative Adversarial Networks. Journal of Digital Imaging. Published online March 13, 2023. doi:https://doi.org/10.1007/s10278-023-00803-2
Roscoe RD, Becker DV, Branaghan RJ, et al. Bridging psychology and engineering to make technology work for people. American Psychologist. 2019;74(3):394-406. doi:https://doi.org/10.1037/amp0000444
Cohen J, Safdi MA, Deal SE, et al. Quality Indicators for Esophagogastroduodenoscopy. Official journal of the American College of Gastroenterology | ACG. 2006;101(4):886. Accessed December 28, 2023. https://journals.lww.com/ajg/citation/2006/04000/quality_indicators_for_esophagogastroduodenoscopy.33.aspx
Ahmed O, Ajani JA, Lee JH. Endoscopic management of esophageal cancer. World Journal of Gastrointestinal Oncology. 2019;11(10):830-841. doi:https://doi.org/10.4251/wjgo.v11.i10.830
Sonnenberg A, Amorosi SL, Lacey MJ, Lieberman DA. Patterns of endoscopy in the United States: analysis of data from the Centers for Medicare and Medicaid Services and the National Endoscopic Database. Gastrointestinal Endoscopy. 2008;67(3):489-496. doi:https://doi.org/10.1016/j.gie.2007.08.041
Ribeiro T. Artificial intelligence and capsule endoscopy: automatic detection of vascular lesions using a convolutional neural network. Annals of Gastroenterology. Published online 2021. doi:https://doi.org/10.20524/aog.2021.0653
Dong Z, Wu L, Mu G, et al. A deep learning-based system for real-time image reporting during esophagogastroduodenoscopy: a multicenter study. Endoscopy. 2022;54(08):771-777. doi:https://doi.org/10.1055/a-1731-9535
Ebigbo A, Palm C, Probst A, et al. A technical review of artificial intelligence as applied to gastrointestinal endoscopy: clarifying the terminology. Endoscopy International Open. 2019;07(12):E1616-E1623. doi:https://doi.org/10.1055/a-1010-5705
Riemann JF, Teufel A. Digital Communication Strategies in Visceral Medicine. Visceral Medicine. 2021;37(6):455-457. doi:https://doi.org/10.1159/000518878
Filipe Vilas-Boas, Ribeiro T, Afonso J, et al. Deep Learning for Automatic Differentiation of Mucinous versus Non-Mucinous Pancreatic Cystic Lesions: A Pilot Study. Diagnostics. 2022;12(9):2041-2041. doi:https://doi.org/10.3390/diagnostics12092041
Ribeiro, T., Saraiva, M M., Ferreira, J., Cardoso, H., Afonso, J., Andrade, P., Parente, M., Jorge, R N., & Macedo, G. (2021, January 1). Artificial intelligence and capsule endoscopy: automatic detection of vascular lesions using a convolutional neural network. https://doi.org/10.20524/aog.2021.0653
Mărginean, C O., Meliţ, L E., & Săsăran, M O. (2022, July 1). Traditional and Modern Diagnostic Approaches in Diagnosing Pediatric Helicobacter pylori Infection. https://doi.org/10.3390/children9070994
Bang, C S., Lee, J J., & Baik, G H. (2021, December 14). Computer-Aided Diagnosis of Gastrointestinal Ulcer and Hemorrhage Using Wireless Capsule Endoscopy: Systematic Review and Diagnostic Test Accuracy Meta-analysis.
Yu, F., Song, E., Liu, H., Zhu, J., & Hung, C. (2019, January 1). Laparoscopic Image-Guided System Based on Multispectral Imaging for the Ureter Detection.
Mascarenhas, M., Ribeiro, T., Afonso, J., Andrade, P., Cardoso, P., Ferreira, J., Cardoso, H., & Macedo, G. (2021, December 18). Deep Learning and Device-Assisted Enteroscopy: Automatic Detection of Gastrointestinal Angioectasia.
Seok, C W. (2020, September 16). Artificial Intelligence for the Prediction of Helicobacter Pylori Infection in Endoscopic Images: Systematic Review and Meta-Analysis Of Diagnostic Test Accuracy.
Kim, J H., Oh, S., Han, S., Keum, J., Kim, K., Chun, J., Youn, Y H., & Park, H. (2022, December 5). An Optimal Artificial Intelligence System for Real-Time Endoscopic Prediction of Invasion Depth in Early Gastric Cancer.
Riemann, J F., & Teufel, A. (2021, January 1). Digital Communication Strategies in Visceral Medicine. https://doi.org/10.1159/000518878
Vilas-Boas, F., Ribeiro, T., Afonso, J., Cardoso, H., Lopes, S., Moutinho-Ribeiro, P., Ferreira, J., Mascarenhas, M., & Macedo, G. (2022, August 24). Deep Learning for Automatic Differentiation of Mucinous versus Non-Mucinous Pancreatic Cystic Lesions: A Pilot Study.
Dong, Z., Wu, L., Mu, G., Zhou, W., Li, Y., Shi, Z., Xia, T., Liu, S., Zhu, Q., Shang, R., Zhang, M., Zhang, L., Xu, M., Zhu, Y., Xiao, T., Chen, T., Li, X., Zhang, C., He, X., . . . Yu, H. (2022, March 10). A deep learning-based system for real-time image reporting during esophagogastroduodenoscopy: a multicenter study. https://doi.org/10.1055/a-1731-9535
Mărginean CO, Meliț LE, Săsăran MO. Traditional and Modern Diagnostic Approaches in Diagnosing Pediatric Helicobacter pylori Infection. Children. 2022;9(7):994. doi:https://doi.org/10.3390/children9070994
Bang CS, Lee JJ, Baik GH. Computer-Aided Diagnosis of Gastrointestinal Ulcer and Hemorrhage Using Wireless Capsule Endoscopy: Systematic Review and Diagnostic Test Accuracy Meta-analysis. Journal of Medical Internet Research. 2021;23(12):e33267. doi:https://doi.org/10.2196/33267
Yu F, Song E, Liu H, Zhu J, Hung C. Laparoscopic Image-Guided System Based on Multispectral Imaging for the Ureter Detection. IEEE Access. 2019;7:3800-3809. doi:https://doi.org/10.1109/access.2018.2889138
Mascarenhas Saraiva M, Ribeiro T, Afonso J, et al. Deep Learning and Device-Assisted Enteroscopy: Automatic Detection of Gastrointestinal Angioectasia. Medicina. 2021;57(12):1378. doi:https://doi.org/10.3390/medicina57121378
Bang CS, Lee JJ, Baik GH. Artificial Intelligence for the Prediction of Helicobacter Pylori Infection in Endoscopic Images: Systematic Review and Meta-Analysis Of Diagnostic Test Accuracy. Journal of Medical Internet Research. 2020;22(9):e21983. doi:https://doi.org/10.2196/21983
Kim JH, Oh SI, Han SY, et al. An Optimal Artificial Intelligence System for Real-Time Endoscopic Prediction of Invasion Depth in Early Gastric Cancer. Cancers. 2022;14(23):6000. doi:https://doi.org/10.3390/cancers14236000
Riemann JF, Teufel A. Digital Communication Strategies in Visceral Medicine. Visceral Medicine. 2021;37(6):455-457. doi:https://doi.org/10.1159/000518878
Fitzgerald RC, di Pietro M, Ragunath K, et al. British Society of Gastroenterology guidelines on the diagnosis and management of Barrett’s oesophagus. Gut. 2013;63(1):7-42. doi:https://doi.org/10.1136/gutjnl-2013-305372
Ali S, Zhou F, Braden B, et al. An objective comparison of detection and segmentation algorithms for artefacts in clinical endoscopy. 2020;10(1). doi:https://doi.org/10.1038/s41598-020-59413-5
Li J, Wang L, Ang T. Artificial intelligence-assisted colonoscopy: a narrative review of current data and clinical applications. Singapore Medical Journal. 2022;63(3):118-124. doi:https://doi.org/10.11622/smedj.2022044
Kumagai Y, Kaiyo Takubo, Kawada K, et al. Endocytoscopic Observation of Esophageal Lesions: Our Own Experience and a Review of the Literature. Diagnostics. 2022;12(9):2222-2222. doi:https://doi.org/10.3390/diagnostics12092222
Misumi Y, Nonaka K, Takeuchi M, et al. Comparison of the Ability of Artificial-Intelligence-Based Computer-Aided Detection (CAD) Systems and Endoscopists to Detect Colorectal Neoplastic Lesions on Endoscopy Video. Journal of Clinical Medicine. 2023;12(14):4840. doi:https://doi.org/10.3390/jcm12144840
Ebigbo A, Palm C, Probst A, et al. A technical review of artificial intelligence as applied to gastrointestinal endoscopy: clarifying the terminology. Endoscopy International Open. 2019;07(12):E1616-E1623. doi:https://doi.org/10.1055/a-1010-5705
Xue P, Qiao YL, Jiang Y. [Application of artificial intelligence in diagnosis of medical endoscope]. Zhonghua Zhong Liu Za Zhi [Chinese Journal of Oncology]. 2018;40(12):890-893. doi:https://doi.org/10.3760/cma.j.issn.0253-3766.2018.12.003
Bang CS, Lee JJ, Baik GH. Computer-Aided Diagnosis of Gastrointestinal Ulcer and Hemorrhage Using Wireless Capsule Endoscopy: Systematic Review and Diagnostic Test Accuracy Meta-analysis. Journal of Medical Internet Research. 2021;23(12):e33267. doi:https://doi.org/10.2196/33267
Yu X, Song Z, Zou S, et al. Artificial Intelligence Assisted Topographic Mapping System for Endoscopic Submucosal Dissection Specimens. Frontiers in Medicine. 2022;9. doi:https://doi.org/10.3389/fmed.2022.822731
Filipe Vilas-Boas, Ribeiro T, Afonso J, et al. Deep Learning for Automatic Differentiation of Mucinous versus Non-Mucinous Pancreatic Cystic Lesions: A Pilot Study. Diagnostics. 2022;12(9):2041-2041. doi:https://doi.org/10.3390/diagnostics12092041
Vilas-Boas, F., Ribeiro, T., Afonso, J., Cardoso, H., Lopes, S., Moutinho-Ribeiro, P., Ferreira, J., Mascarenhas, M., & Macedo, G. (2022, August 24). Deep Learning for Automatic Differentiation of Mucinous versus Non-Mucinous Pancreatic Cystic Lesions: A Pilot Study. https://doi.org/10.3390/diagnostics12092041
Spadaccini, M., Massimi, D., Mori, Y., Alfarone, L., Fugazza, A., Maselli, R., Facciorusso, A., Hassan, C., & Repici, A. (2023, March 14). Artificial Intelligence-Aided Endoscopy and Colorectal Cancer Screening. https://doi.org/10.3390/diagnostics13061102
Zhang, M., Zhu, C., Wang, Y., Kong, Z., Hua, Y., Zhang, W., Si, X., Ye, B., Xü, X., Li, L., Heng, D., Liu, B., Song, T., Wu, J., Dang, Y., & Zhang, G. (2021, June 1). Differential diagnosis for esophageal protruded lesions using a deep convolution neural network in endoscopic images.
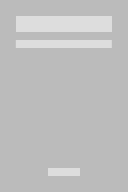
Opublikowane
Licencja

Utwór dostępny jest na licencji Creative Commons Uznanie autorstwa – Użycie niekomercyjne – Bez utworów zależnych 4.0 Międzynarodowe.