Sztuczna inteligencja w profilaktyce raka jelita grubego
Słowa kluczowe:
rak jelita grubego, profilaktyka, sztuczna inteligencjaStreszczenie
Sztuczna inteligencja jest zdolnością systemu do prawidłowego interpretowania danych pochodzących z zewnętrznych źródeł, nauki na ich podstawie oraz wykorzystywania tej wiedzy, aby wykonywać określone zadania i osiągać cele poprzez elastyczne dostosowanie, przez co wykazuje zachowania częściowo inteligentne. Narzędzie to znajduje zastosowania w wielu aspektach życia codziennego, przemyśle jak i w wielu dziedzinach nauki, także w medycynie. Jednym z zastosowań sztucznej inteligencji w onkologii, które zostanie omówione w niniejszym rozdziale, jest profilaktyka i diagnostyka raka jelita grubego, który jest drugim co do częstości występowania nowotworem złośliwym na świecie a wczesna diagnoza i zapobieganie są kluczowe do ograniczenia częstości występowania tego nowotworu. Rak jelita grubego charakteryzuje się często bezobjawowym przebiegiem, późnym wykrywaniem i wysoką śmiertelnością. Programy sztucznej inteligencji znalazły zastosowanie w wielu badaniach przesiewowych będących podstawą profilaktyki nowotworu złośliwego jelita grubego, znacznie zwiększając ich skuteczność i wartość diagnostyczną. Algorytmy te umożliwiają, poprzez porównywanie i analizę danych pacjenta oraz wyników jego badań, skuteczne i zautomatyzowane wykrywanie patologii, dając szanse ich wykrycia jeszcze przed przekształceniem się w zmiany złośliwe.
Bibliografia
Sánchez-Guillén L, Arroyo A. Immunonutrition in patients with colon cancer. Immunotherapy. 2020;12(1):5-8. doi:10.2217/imt-2019-0179.
Baidoun F, Elshiwy K, Elkeraie Y, et al. Colorectal Cancer Epidemiology: Recent Trends and Impact on Outcomes. Curr Drug Targets. 2021;22(9):998-1009. doi:10.2174/1389450121999201117115717
Thanikachalam K, Khan G. Colorectal Cancer and Nutrition. Nutrients. 2019;11(1):164. Published 2019 Jan 14. doi:10.3390/nu11010164
Benson AB, Venook AP, Al-Hawary MM, et al. Colon Cancer, Version 2.2021, NCCN Clinical Practice Guidelines in Oncology. J Natl Compr Canc Netw. 2021;19(3):329-359. Published 2021 Mar 2. doi:10.6004/jnccn.2021.0012
Chen M, Decary M. Artificial intelligence in healthcare: An essential guide for health leaders. Healthc Manage Forum. 2020;33(1):10-18. doi:10.1177/0840470419873123
Gupta R, Srivastava D, Sahu M, Tiwari S, Ambasta RK, Kumar P. Artificial intelligence to deep learning: machine intelligence approach for drug discovery. Mol Divers. 2021;25(3):1315-1360. doi:10.1007/s11030-021-10217-3
Mintz Y, Brodie R. Introduction to artificial intelligence in medicine. Minim Invasive Ther Allied Technol. 2019;28(2):73-81. doi:10.1080/13645706.2019.1575882
Ruffle JK, Farmer AD, Aziz Q. Artificial Intelligence-Assisted Gastroenterology- Promises and Pitfalls. Am J Gastroenterol. 2019;114(3):422-428. doi:10.1038/s41395-018-0268-4
Hamet P, Tremblay J. Artificial intelligence in medicine. Metabolism. 2017;69S:S36-S40. doi:10.1016/j.metabol.2017.01.011
Maida M, Macaluso FS, Ianiro G, et al. Screening of colorectal cancer: present and future. Expert Rev Anticancer Ther. 2017;17(12):1131-1146. doi:10.1080/14737140.2017.1392243
Mandel JS, Bond JH, Church TR, et al. Reducing mortality from colorectal cancer by screening for fecal occult blood. Minnesota Colon Cancer Control Study [published correction appears in N Engl J Med 1993 Aug 26;329(9):672]. N Engl J Med. 1993;328(19):1365-1371. doi:10.1056/NEJM199305133281901
Hewitson P, Glasziou P, Irwig L, Towler B, Watson E. Screening for colorectal cancer using the faecal occult blood test, Hemoccult. Cochrane Database Syst Rev. 2007;2007(1):CD001216. Published 2007 Jan 24. doi:10.1002/14651858.CD001216.pub2
Smith RA, Andrews KS, Brooks D, et al. Cancer screening in the United States, 2019: A review of current American Cancer Society guidelines and current issues in cancer screening. CA: A Cancer Journal for Clinicians. 2019;69(3):184-210. doi:https://doi.org/10.3322/caac.21557
Issa IA, Noureddine M. Colorectal cancer screening: An updated review of the available options. World Journal of Gastroenterology. 2017;23(28):5086. doi:https://doi.org/10.3748/wjg.v23.i28.5086
Mitsala A, Tsalikidis C, Pitiakoudis M, Simopoulos C, Tsaroucha AK. Artificial Intelligence in Colorectal Cancer Screening, Diagnosis and Treatment. A New Era. Curr Oncol. 2021;28(3):1581-1607. Published 2021 Apr 23. doi:10.3390/curroncol28030149
Hilsden RJ, Heitman SJ, Mizrahi B, Narod SA, Goshen R. Prediction of findings at screening colonoscopy using a machine learning algorithm based on complete blood counts (ColonFlag). Cheah PY, ed. PLOS ONE. 2018;13(11):e0207848. doi:https://doi.org/10.1371/journal.pone.0207848
Kinar Y, Kalkstein N, Akiva P, et al. Development and validation of a predictive model for detection of colorectal cancer in primary care by analysis of complete blood counts: a binational retrospective study. Journal of the American Medical Informatics Association. 2016;23(5):879-890. doi:https://doi.org/10.1093/jamia/ocv195
Hornbrook MC, Goshen R, Choman E, et al. Early Colorectal Cancer Detected by Machine Learning Model Using Gender, Age, and Complete Blood Count Data. Digestive Diseases and Sciences. 2017;62(10):2719-2727. doi:https://doi.org/10.1007/s10620-017-4722-8
Bressler B, Paszat LF, Chen Z, Rothwell DM, Vinden C, Rabeneck L. Rates of New or Missed Colorectal Cancers After Colonoscopy and Their Risk Factors: A Population-Based Analysis. Gastroenterology. 2007;132(1):96-102. doi:https://doi.org/10.1053/j.gastro.2006.10.027
Morris EJA, Rutter MD, Finan PJ, Thomas JD, Valori R. Post-colonoscopy colorectal cancer (PCCRC) rates vary considerably depending on the method used to calculate them: a retrospective observational population-based study of PCCRC in the English National Health Service. Gut. 2014;64(8):1248-1256. doi:https://doi.org/10.1136/gutjnl-2014-308362
Corley DA, Jensen CD, Marks AR, et al. Adenoma Detection Rate and Risk of Colorectal Cancer and Death. New England Journal of Medicine. 2014;370(14):1298-1306. doi:https://doi.org/10.1056/nejmoa1309086
Ahn SB, Han DS, Bae JH, Byun TJ, Kim JP, Eun CS. The Miss Rate for Colorectal Adenoma Determined by Quality-Adjusted, Back-to-Back Colonoscopies. Gut and Liver. 2012;6(1):64-70. doi:https://doi.org/10.5009/gnl.2012.6.1.64
van Rijn JC, Reitsma JB, Stoker J, Bossuyt PM, van Deventer SJ, Dekker E. Polyp miss rate determined by tandem colonoscopy: a systematic review. Am J Gastroenterol. 2006;101(2):343-350. doi:10.1111/j.1572-0241.2006.00390.x
Lee CK, Park DI, Lee SH, et al. Participation by experienced endoscopy nurses increases the detection rate of colon polyps during a screening colonoscopy: a multicenter, prospective, randomized study. Gastrointest Endosc. 2011;74(5):1094-1102. doi:10.1016/j.gie.2011.06.033
Mori Y, Kudo S, Berzin T, Misawa M, Takeda K. Computer-aided diagnosis for colonoscopy. Endoscopy. 2017;49(08):813-819. doi:https://doi.org/10.1055/s-0043-109430
Shin HC, Roth HR, Gao M, et al. Deep Convolutional Neural Networks for Computer-Aided Detection: CNN Architectures, Dataset Characteristics and Transfer Learning. IEEE Transactions on Medical Imaging. 2016;35(5):1285-1298. doi:https://doi.org/10.1109/tmi.2016.2528162
Wang P, Berzin TM, Glissen Brown JR, et al. Real-time automatic detection system increases colonoscopic polyp and adenoma detection rates: a prospective randomised controlled study. Gut. 2019;68(10):1813-1819. doi:https://doi.org/10.1136/gutjnl-2018-317500
Eliakim R, Fireman Z, Gralnek IM, et al. Evaluation of the PillCam Colon capsule in the detection of colonic pathology: results of the first multicenter, prospective, comparative study. Endoscopy. 2006;38(10):963-970. doi:10.1055/s-2006-944832
Blanes-Vidal V, Baatrup G, Nadimi ES. Addressing priority challenges in the detection and assessment of colorectal polyps from capsule endoscopy and colonoscopy in colorectal cancer screening using machine learning. Acta Oncol. 2019;58(sup1):S29-S36. doi:10.1080/0284186X.2019.1584404
East JE, Vleugels JL, Roelandt P, et al. Advanced endoscopic imaging: European Society of Gastrointestinal Endoscopy (ESGE) Technology Review. Endoscopy. 2016;48(11):1029-1045. doi:10.1055/s-0042-118087
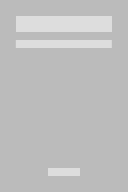
Zapowiedzi
Licencja

Utwór dostępny jest na licencji Creative Commons Uznanie autorstwa – Użycie niekomercyjne – Bez utworów zależnych 4.0 Międzynarodowe.