Zastosowanie sztucznej inteligencji w analizie obrazów medycznych
Słowa kluczowe:
sztuczna inteligencja, uczenie maszynowe, sieci neuronowe, AIStreszczenie
Rozwój sztucznej inteligencji (AI) i technologii cyfrowych przyniósł rewolucję w dziedzinie medycyny, szczególnie w analizie obrazów medycznych. Tradycyjnie, ich interpretacja była zależna od umiejętności i doświadczenia lekarzy, co wprowadzało pewien stopień subiektywizmu i ograniczało efektywność diagnozowania. Jednak rozwój technik sztucznej inteligencji, takich jak głębokie sieci neuronowe (DNN), konwolucyjne sieci neuronowe (CNN), czy uczenie maszynowe umożliwiają automatyczną analizę, diagnozowanie i klasyfikację obrazów medycznych. W dalszej części pracy przedstawione zostanie aktualny stan wiedzy na temat analizy obrazów medycznych za pomocą AI, w tym najnowszych badań, technik, metod i wyników osiągniętych w tej dziedzinie.
Bibliografia
Referencje
Khafaji MA, Safhi MA, Albadawi RH, Al-Amoudi SO, Shehata SS, Toonsi F. Artificial intelligence in radiology: Are Saudi residents ready, prepared, and knowledgeable?. Saudi Med J. 2022;43(1):53-60. doi:10.15537/smj.2022.43.1.20210337
Esteva A, Kuprel B, Novoa RA, et al. Dermatologist-level classification of skin cancer with deep neural networks [published correction appears in Nature. 2017 Jun 28;546(7660):686]. Nature. 2017;542(7639):115-118. doi:10.1038/nature21056
Litjens G, Kooi T, Bejnordi BE, et al. A survey on deep learning in medical image analysis. Med Image Anal. 2017;42:60-88. doi:10.1016/j.media.2017.07.005
LeCun Y, Bengio Y, Hinton G. Deep learning. Nature. 2015;521(7553):436-444. doi:10.1038/nature14539
Iqbal S, N. Qureshi A, Li J, Mahmood T. On the Analyses of Medical Images Using Traditional Machine Learning Techniques and Convolutional Neural Networks. Arch Comput Methods Eng. 2023;30(5):3173-3233. doi:10.1007/s11831-023-09899-9
Dhaka VS, Meena SV, Rani G, et al. A Survey of Deep Convolutional Neural Networks Applied for Prediction of Plant Leaf Diseases. Sensors (Basel). 2021;21(14):4749. Published 2021 Jul 12. doi:10.3390/s21144749
Onyema EM, Almuzaini KK, Onu FU, et al. Prospects and Challenges of Using Machine Learning for Academic Forecasting. Comput Intell Neurosci. 2022;2022:5624475. Published 2022 Jun 17. doi:10.1155/2022/5624475
Jayatilake SMDAC, Ganegoda GU. Involvement of Machine Learning Tools in Healthcare Decision Making. J Healthc Eng. 2021;2021:6679512. Published 2021 Jan 27. doi:10.1155/2021/6679512
Sabry F, Eltaras T, Labda W, Alzoubi K, Malluhi Q. Machine Learning for Healthcare Wearable Devices: The Big Picture. J Healthc Eng. 2022;2022:4653923. Published 2022 Apr 18. doi:10.1155/2022/4653923
Yi J, Kang HK, Kwon JH, et al. Technology trends and applications of deep learning in ultrasonography: image quality enhancement, diagnostic support, and improving workflow efficiency. Ultrasonography. 2021;40(1):7-22. doi:10.14366/usg.20102
Akkus, Z.; Cai, J.; Boonrod, A.; Zeinoddini, A.; Weston, A.D.; Philbrick, K.A.; Erickson, B.J. A Survey of Deep-LearningApplications in Ultrasound: Artificial Intelligence-Powered Ultrasound for Improving Clinical Workflow. J. Am. Coll. Radiol.2019,16, 1318–1328.
Komatsu M, Sakai A, Dozen A, et al. Towards Clinical Application of Artificial Intelligence in Ultrasound Imaging. Biomedicines. 2021;9(7):720. Published 2021 Jun 23. doi:10.3390/biomedicines9070720
Sung CK, Lee KH, Kim SH. Evaluation of factors influencing arterial Doppler waveforms in an in vitro flow phantom. Ultrasonography. 2017;36(1):39-52. doi:10.14366/usg.15055
Guo Z, Xie J, Wan Y, et al. A review of the current state of the computer-aided diagnosis (CAD) systems for breast cancer diagnosis. Open Life Sci. 2022;17(1):1600-1611. Published 2022 Dec 9. doi:10.1515/biol-2022-0517
Shaheen F, Chowdry N, Gojwari T, Wani AI, Khan S. Role of cervical ultrasonography in primary hyperparathyroidism. Indian J Radiol Imaging. 2008;18(4):302-305. doi:10.4103/0971-3026.43846
Jassal K, Koohestani A, Kiu A, et al. Artificial Intelligence for Pre-operative Diagnosis of Malignant Thyroid Nodules Based on Sonographic Features and Cytology Category. World J Surg. 2023;47(2):330-339. doi:10.1007/s00268-022-06798-1
Li H, Bhatt M, Qu Z, et al. Deep learning in ultrasound elastography imaging: A review. Med Phys. 2022;49(9):5993-6018. doi:10.1002/mp.15856
Rajpurkar P, Irvin J, Ball RL, et al. Deep learning for chest radiograph diagnosis: A retrospective comparison of the CheXNeXt algorithm to practicing radiologists. PLoS Med. 2018;15(11):e1002686. Published 2018 Nov 20. doi:10.1371/journal.pmed.1002686
Gulshan V, Peng L, Coram M, et al. Development and Validation of a Deep Learning Algorithm for Detection of Diabetic Retinopathy in Retinal Fundus Photographs. JAMA. 2016;316(22):2402–2410. doi:10.1001/jama.2016.17216
Lakhani P, Sundaram B. Deep Learning at Chest Radiography: Automated Classification of Pulmonary Tuberculosis by Using Convolutional Neural Networks. Radiology. 2017;284(2):574-582. doi:10.1148/radiol.2017162326
Hashmi MF, Katiyar S, Keskar AG, Bokde ND, Geem ZW. Efficient Pneumonia Detection in Chest Xray Images Using Deep Transfer Learning. Diagnostics (Basel). 2020;10(6):417. Published 2020 Jun 19. doi:10.3390/diagnostics10060417
Yan K, Wang X, Lu L, Summers RM. DeepLesion: automated mining of large-scale lesion annotations and universal lesion detection with deep learning. J Med Imaging (Bellingham). 2018;5(3):036501. doi:10.1117/1.JMI.5.3.036501
Gore JC. Artificial intelligence in medical imaging. Magn Reson Imaging. 2020;68:A1-A4. doi:10.1016/j.mri.2019.12.006
De Nunzio G, Tommasi E, Agrusti A, et al. Automatic lung segmentation in CT images with accurate handling of the hilar region. J Digit Imaging. 2011;24(1):11-27. doi:10.1007/s10278-009-9229-1
Lee JG, Jun S, Cho YW, et al. Deep Learning in Medical Imaging: General Overview. Korean J Radiol. 2017;18(4):570-584. doi:10.3348/kjr.2017.18.4.570
Paiva OA, Prevedello LM. The potential impact of artificial intelligence in radiology. Radiol Bras. 2017;50(5):V-VI. doi:10.1590/0100-3984.2017.50.5e1
Johnson KB, Wei WQ, Weeraratne D, et al. Precision Medicine, AI, and the Future of Personalized Health Care. Clin Transl Sci. 2021;14(1):86-93. doi:10.1111/cts.12884
Miotto R, Wang F, Wang S, Jiang X, Dudley JT. Deep learning for healthcare: review, opportunities and challenges. Brief Bioinform. 2018;19(6):1236-1246. doi:10.1093/bib/bbx044
Waller J, O'Connor A, Rafaat E, et al. Applications and challenges of artificial intelligence in diagnostic and interventional radiology. Pol J Radiol. 2022;87:e113-e117. Published 2022 Feb 25. doi:10.5114/pjr.2022.113531
Brady AP, Neri E. Artificial Intelligence in Radiology-Ethical Considerations. Diagnostics (Basel). 2020;10(4):231. Published 2020 Apr 17. doi:10.3390/diagnostics10040231
Kulkarni V, Gawali M, Kharat A. Key Technology Considerations in Developing and Deploying Machine Learning Models in Clinical Radiology Practice. JMIR Med Inform. 2021;9(9):e28776. Published 2021 Sep 9. doi:10.2196/28776
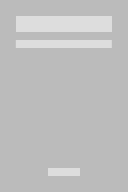
Zapowiedzi
Licencja

Utwór dostępny jest na licencji Creative Commons Uznanie autorstwa – Użycie niekomercyjne – Bez utworów zależnych 4.0 Międzynarodowe.