Zastosowanie sztucznej inteligencji w immunoterapii nowotworów
Słowa kluczowe:
sztuczna inteligencja, nowotwory, immunoterapia, TMEStreszczenie
Nowotwory są narastającym problemem w Polsce i na świecie. Do jego rozwiązania możliwe jest zastosowanie sztucznej inteligencji (AI), która znalazła swoje zastosowanie w diagnostyce nowotworów oraz projektowaniu immunoterapii chorób nowotworowych. Szczególną zdolnością wykazywaną przez AI jest zdolność do precyzyjnego opisania mikrośrodowiska guza (TME), które pełni istotną rolę w jego rozprzestrzenianiu się, a także w odpowiedzi na immunoterapię. W niektórych obszarach, związanych z onkologią i immunoterapią, AI może działać z większą skutecznością niż człowiek, jednocześnie wykonując te zadania w krótszym czasie niż on. Zastosowanie technik AI w onkologii udziela wiele wskazówek diagnostycznych i terapeutycznych, a także pozwala na prognozę wyleczenia i przeżycia chorego. AI ułatwia również projektowanie nowych leków, co przyspiesza rozwój medycyny.
Bibliografia
Didkowska J, Wojciechowska U, Michalek IM, Caetano Dos Santos FL. Cancer incidence and mortality in Poland in 2019. Sci Rep. 2022;12(1):10875. Published 2022 Jun 27. doi:10.1038/s41598-022-14779-6
Bray F, Ferlay J, Soerjomataram I, Siegel RL, Torre LA, Jemal A. Global cancer statistics 2018: GLOBOCAN estimates of incidence and mortality worldwide for 36 cancers in 185 countries [published correction appears in CA Cancer J Clin. 2020 Jul;70(4):313]. CA Cancer J Clin. 2018;68(6):394-424. doi:10.3322/caac.21492
Golemis EA, Scheet P, Beck TN, et al. Molecular mechanisms of the preventable causes of cancer in the United States. Genes Dev. 2018;32(13-14):868-902. doi:10.1101/gad.314849.118
Ramesh AN, Kambhampati C, Monson JR, Drew PJ. Artificial intelligence in medicine. Ann R Coll Surg Engl. 2004;86(5):334-338. doi:10.1308/147870804290
Hamet P, Tremblay J. Artificial intelligence in medicine. Metabolism. 2017;69S:S36-S40. doi:10.1016/j.metabol.2017.01.011
Fu Y, Liu S, Zeng S, Shen H. From bench to bed: the tumor immune microenvironment and current immunotherapeutic strategies for hepatocellular carcinoma. J Exp Clin Cancer Res. 2019;38(1):396. Published 2019 Sep 9. doi:10.1186/s13046-019-1396-4
Wahyuhadi J, Immadoel Haq IB, Arifianto MR, et al. Active Immunotherapy for Glioblastoma Treatment: A Systematic Review and Meta-Analysis. Cancer Control. 2022;29:10732748221079474. doi:10.1177/10732748221079474
Mitra S, Li G, Harsh GR 4th. Passive antibody-mediated immunotherapy for the treatment of malignant gliomas. Neurosurg Clin N Am. 2010;21(1):67-76. doi:10.1016/j.nec.2009.08.010
Restifo NP, Dudley ME, Rosenberg SA. Adoptive immunotherapy for cancer: harnessing the T cell response. Nat Rev Immunol. 2012;12(4):269-281. Published 2012 Mar 22. doi:10.1038/nri3191
Nishino M, Ramaiya NH, Hatabu H, Hodi FS. Monitoring immune-checkpoint blockade: response evaluation and biomarker development. Nat Rev Clin Oncol. 2017;14(11):655-668. doi:10.1038/nrclinonc.2017.88
Soularue E, Lepage P, Colombel JF, et al. Enterocolitis due to immune checkpoint inhibitors: a systematic review. Gut. 2018;67(11):2056-2067. doi:10.1136/gutjnl-2018-316948
Angell H, Galon J. From the immune contexture to the Immunoscore: the role of prognostic and predictive immune markers in cancer. Curr Opin Immunol. 2013;25(2):261-267. doi:10.1016/j.coi.2013.03.004
Mekki A, Dercle L, Lichtenstein P, et al. Machine learning defined diagnostic criteria for differentiating pituitary metastasis from autoimmune hypophysitis in patients undergoing immune checkpoint blockade therapy. Eur J Cancer. 2019;119:44-56. doi:10.1016/j.ejca.2019.06.020
Martins J, Magalhães C, Rocha M, Osório NS. Machine Learning-Enhanced T Cell Neoepitope Discovery for Immunotherapy Design. Cancer Inform. 2019;18:1176935119852081. Published 2019 May 23. doi:10.1177/1176935119852081
Langs G, Röhrich S, Hofmanninger J, et al. Machine learning: from radiomics to discovery and routine. Maschinelles Lernen: von Radiomics zu Forschung und Routine. Radiologe. 2018;58(Suppl 1):1-6. doi:10.1007/s00117-018-0407-3
Kleppe A, Skrede OJ, De Raedt S, Liestøl K, Kerr DJ, Danielsen HE. Designing deep learning studies in cancer diagnostics. Nat Rev Cancer. 2021;21(3):199-211. doi:10.1038/s41568-020-00327-9
Hosny A, Parmar C, Quackenbush J, Schwartz LH, Aerts HJWL. Artificial intelligence in radiology. Nat Rev Cancer. 2018;18(8):500-510. doi:10.1038/s41568-018-0016-5
Kooi T, Litjens G, van Ginneken B, et al. Large scale deep learning for computer aided detection of mammographic lesions. Med Image Anal. 2017;35:303-312. doi:10.1016/j.media.2016.07.007
Trebeschi S, Drago SG, Birkbak NJ, et al. Predicting response to cancer immunotherapy using noninvasive radiomic biomarkers. Ann Oncol. 2019;30(6):998-1004. doi:10.1093/annonc/mdz108
Sirinukunwattana K, Ahmed Raza SE, Yee-Wah Tsang, Snead DR, Cree IA, Rajpoot NM. Locality Sensitive Deep Learning for Detection and Classification of Nuclei in Routine Colon Cancer Histology Images. IEEE Trans Med Imaging. 2016;35(5):1196-1206. doi:10.1109/TMI.2016.2525803
Kather JN, Pearson AT, Halama N, et al. Deep learning can predict microsatellite instability directly from histology in gastrointestinal cancer. Nat Med. 2019;25(7):1054-1056. doi:10.1038/s41591-019-0462-y
Le DT, Uram JN, Wang H, et al. PD-1 Blockade in Tumors with Mismatch-Repair Deficiency. N Engl J Med. 2015;372(26):2509-2520. doi:10.1056/NEJMoa1500596
Zaitsev A, Chelushkin M, Dyikanov D, et al. Precise reconstruction of the TME using bulk RNA-seq and a machine learning algorithm trained on artificial transcriptomes. Cancer Cell. 2022;40(8):879-894.e16. doi:10.1016/j.ccell.2022.07.006
Kuksin M, Morel D, Aglave M, et al. Applications of single-cell and bulk RNA sequencing in onco-immunology. Eur J Cancer. 2021;149:193-210. doi:10.1016/j.ejca.2021.03.005
Xiao Y, Yu D. Tumor microenvironment as a therapeutic target in cancer. Pharmacol Ther. 2021;221:107753. doi:10.1016/j.pharmthera.2020.107753
Ji RR, Chasalow SD, Wang L, et al. An immune-active tumor microenvironment favors clinical response to ipilimumab. Cancer Immunol Immunother. 2012;61(7):1019-1031. doi:10.1007/s00262-011-1172-6
Ribas A, Wolchok JD. Cancer immunotherapy using checkpoint blockade. Science. 2018;359(6382):1350-1355. doi:10.1126/science.aar4060
Postow MA, Callahan MK, Wolchok JD. Immune Checkpoint Blockade in Cancer Therapy. J Clin Oncol. 2015;33(17):1974-1982. doi:10.1200/JCO.2014.59.4358
Kalluri R. The biology and function of fibroblasts in cancer. Nat Rev Cancer. 2016;16(9):582-598. doi:10.1038/nrc.2016.73
Orimo A, Gupta PB, Sgroi DC, et al. Stromal fibroblasts present in invasive human breast carcinomas promote tumor growth and angiogenesis through elevated SDF-1/CXCL12 secretion. Cell. 2005;121(3):335-348. doi:10.1016/j.cell.2005.02.034
Lakins MA, Ghorani E, Munir H, Martins CP, Shields JD. Cancer-associated fibroblasts induce antigen-specific deletion of CD8 + T Cells to protect tumour cells. Nat Commun. 2018;9(1):948. Published 2018 Mar 5. doi:10.1038/s41467-018-03347-0
DeNardo DG, Ruffell B. Macrophages as regulators of tumour immunity and immunotherapy. Nat Rev Immunol. 2019;19(6):369-382. doi:10.1038/s41577-019-0127-6
Ruffell B, Coussens LM. Macrophages and therapeutic resistance in cancer. Cancer Cell. 2015;27(4):462-472. doi:10.1016/j.ccell.2015.02.015
Chen FH, Chiang CS, Wang CC, et al. Radiotherapy decreases vascular density and causes hypoxia with macrophage aggregation in TRAMP-C1 prostate tumors. Clin Cancer Res. 2009;15(5):1721-1729. doi:10.1158/1078-0432.CCR-08-1471
Hughes R, Qian BZ, Rowan C, et al. Perivascular M2 Macrophages Stimulate Tumor Relapse after Chemotherapy. Cancer Res. 2015;75(17):3479-3491. doi:10.1158/0008-5472.CAN-14-3587
Yarchoan M, Johnson BA 3rd, Lutz ER, Laheru DA, Jaffee EM. Targeting neoantigens to augment antitumour immunity [published correction appears in Nat Rev Cancer. 2017 Aug 24;17 (9):569]. Nat Rev Cancer. 2017;17(4):209-222. doi:10.1038/nrc.2016.154
Tran E, Ahmadzadeh M, Lu YC, et al. Immunogenicity of somatic mutations in human gastrointestinal cancers. Science. 2015;350(6266):1387-1390. doi:10.1126/science.aad1253
Bjerregaard AM, Nielsen M, Hadrup SR, Szallasi Z, Eklund AC. MuPeXI: prediction of neo-epitopes from tumor sequencing data. Cancer Immunol Immunother. 2017;66(9):1123-1130. doi:10.1007/s00262-017-2001-3
Bulik-Sullivan B, Busby J, Palmer CD, et al. Deep learning using tumor HLA peptide mass spectrometry datasets improves neoantigen identification [published online ahead of print, 2018 Dec 17]. Nat Biotechnol. 2018;10.1038/nbt.4313. doi:10.1038/nbt.4313
de Sousa E, Lérias JR, Beltran A, et al. Targeting Neoepitopes to Treat Solid Malignancies: Immunosurgery. Front Immunol. 2021;12:592031. Published 2021 Jul 15. doi:10.3389/fimmu.2021.592031
Liu G, Zeng H, Mueller J, et al. Antibody complementarity determining region design using high-capacity machine learning. Bioinformatics. 2020;36(7):2126-2133. doi:10.1093/bioinformatics/btz895
Weitzner BD, Dunbrack RL Jr, Gray JJ. The origin of CDR H3 structural diversity. Structure. 2015;23(2):302-311. doi:10.1016/j.str.2014.11.010
Chiu ML, Goulet DR, Teplyakov A, Gilliland GL. Antibody Structure and Function: The Basis for Engineering Therapeutics. Antibodies (Basel). 2019;8(4):55. Published 2019 Dec 3. doi:10.3390/antib8040055
Akpinaroglu D, Ruffolo JA, Mahajan SP, Gray JJ. Simultaneous prediction of antibody backbone and side-chain conformations with deep learning. PLoS One. 2022;17(6):e0258173. Published 2022 Jun 15. doi:10.1371/journal.pone.0258173
Feng J, Jiang M, Shih J, Chai Q. Antibody apparent solubility prediction from sequence by transfer learning. iScience. 2022;25(10):105173. Published 2022 Sep 22. doi:10.1016/j.isci.2022.105173
Agrawal NJ, Helk B, Kumar S, et al. Computational tool for the early screening of monoclonal antibodies for their viscosities. MAbs. 2016;8(1):43-48. doi:10.1080/19420862.2015.1099773
Prihoda D, Maamary J, Waight A, et al. BioPhi: A platform for antibody design, humanization, and humanness evaluation based on natural antibody repertoires and deep learning. MAbs. 2022;14(1):2020203. doi:10.1080/19420862.2021.2020203
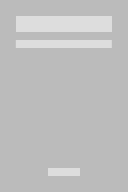
Opublikowane
Licencja

Utwór dostępny jest na licencji Creative Commons Uznanie autorstwa – Użycie niekomercyjne – Bez utworów zależnych 4.0 Międzynarodowe.