Zastosowanie Sztucznej Inteligencji w diagnostyce Retinopatii Cukrzycowej
Słowa kluczowe:
retinopatia cukrzycowa, SI, uczenie głębokieStreszczenie
Retinopatia cukrzycowa stanowi jedno z najczęstszych powikłań cukrzycy i należy do głównych przyczyn utraty wzroku w populacji powyżej 50 roku życia. Najskuteczniejszą metodą zahamowania postępów choroby i uratowania wzroku pacjenta jest wczesny i szybki proces diagnostyczny, pozwalający na wdrożenie odpowiedniej terapii, jednak szeroko zakrojone badania przesiewowe wymagają znacznych nakładów finansowych i obecności wykwalifikowanego personelu medycznego. Odpowiedzią na te trudności może być zastosowanie programów do automatycznej diagnostyki opartych o technologię uczenia głębokiego i konwolucyjne sieci neuronowe. W ostatnich latach pojawia się coraz więcej algorytmów AI, które cechuje wysoka czułość i specyficzność, jednak do dalszego rozwoju systemów automatycznej diagnostyki potrzeba dużej liczby publicznie dostępnych baz zdjęć dna oka pacjentów z retinopatią cukrzycową. W świetle najnowszych odkryć naukowych dotyczących patofizjologii choroby należy również zadać sobie pytanie, czy podobne algorytmy rzeczywiście okażą się użyteczną i skuteczną metodą diagnostyczną w perspektywie najbliższych lat.
Rozdziały
-
Zastosowanie Sztucznej Inteligencji w diagnostyce Retinopatii Cukrzycowej
Bibliografia
Teo ZL, Tham YC, Yu M, et al. Global Prevalence of Diabetic Retinopathy and Projection of Burden Through 2045. Ophthalmology. 2021. doi:10.1016/j.ophtha.2021.04.027
Saeedi P, Petersohn I, Salpea P, et al. Global and Regional Diabetes Prevalence Estimates for 2019 and Projections for 2030 and 2045: Results from the International Diabetes Dederation Diabetes Atlas, 9th edition. Diabetes Research and Clinical Practice. 2019;157:107843. doi:10.1016/j.diabres.2019.107843
Safi H, Safi S, Hafezi-Moghadam A, Ahmadieh H. Early Detection of Diabetic Retinopathy. Survey of Ophthalmology. 2018;63(5):601-608. doi:10.1016/j.survophthal.2018.04.003
Grzybowski A, Brona P, Lim G, et al. Artificial Intelligence for Diabetic Retinopathy Screening: A Review. Eye. 2019;34(3):451-460. doi:10.1038/s41433-019-0566-0
Cheung N, Mitchell P, Wong TY. Diabetic Retinopathy. The Lancet. 2010;376(9735):124-136. doi:10.1016/s0140-6736(09)62124-3
Lechner J, O'Leary OE, Stitt AW. The Pathology Associated with Diabetic Retinopathy. Vision Research. 2017;139:7-14. doi:10.1016/j.visres.2017.04.003
Wolter JR. Diabetic Retinopathy. American Journal of Ophthalmology. 1961;51(5):1123/251-1141/269. doi:10.1016/0002-9394(61)91802-5
Ji L, Tian H, Webster KA, Li W. Neurovascular Regulation in Diabetic Retinopathy and Emerging Therapies. Cellular and Molecular Life Sciences. 2021;78(16):5977-5985. doi:10.1007/s00018-021-03893-9
Moran EP, Wang Z, Chen J, Sapieha P, Smith LE, Ma JX. Neurovascular Cross Talk in Diabetic Retinopathy: Pathophysiological Roles and Therapeutic Implications. American Journal of Physiology-Heart and Circulatory Physiology. 2016;311(3):H738—H749. doi:10.1152/ajpheart.00005.2016
Wong TY, Sun J, Kawasaki R, et al. Guidelines on Diabetic Eye Care. Ophthalmology. 2018;125(10), 1608–1622. https://doi.org/10.1016/j.ophtha.2018.04.007
Vujosevic S, Aldington SJ, Silva P, Hernández C, Scanlon P, Peto T, Simó R. Screening for Diabetic Retinopathy: New Perspectives and Challenges. The Lancet Diabetes & Endocrinology. 2020;8(4), 337–347. https://doi.org/10.1016/s2213-8587(19)30411-5
Ting DSW, Yi PH, Hui F. Clinical Applicability of Deep Learning System in Detecting Tuberculosis with Chest Radiography. Radiology, 2018;286(2), 729–731. https://doi.org/10.1148/radiol.2017172407
Esteva A, Kuprel B, Novoa RA, et al. Dermatologist-Level Classification of Skin Cancer with Deep Neural Networks. Nature. 2017;542(7639), 115–118. https://doi.org/10.1038/nature21056
Ehteshami Bejnordi B, Veta M, Johannes van Diest, et al. (2017). Diagnostic Assessment of Deep Learning Algorithms for Detection of Lymph Node Metastases in Women with Breast Cancer. Jama. 2017;318(22), 2199. https://doi.org/10.1001/jama.2017.14585
Ting DSW, Pasquale LR, Peng L, et al. Artificial intelligence and deep learning in ophthalmology. British Journal of Ophthalmology. 2018;103(2), 167–175. https://doi.org/10.1136/bjophthalmol-2018-313173
Moraru A, Costin D, Moraru R, Branisteanu D. Artificial Intelligence and Deep Learning in Ophthalmology - Present and Future (Review). Exp Ther Med. 2020. doi:10.3892/etm.2020.9118
Li JP, Liu H, Ting DS, et al. Digital Technology, Tele-Medicine and Artificial Intelligence in Ophthalmology: A Global Perspective. Prog Retin Eye Res. 2020:100900. doi:10.1016/j.preteyeres.2020.100900
Stahlschmidt SR, Ulfenborg B, Synnergren J. Multimodal Deep Learning for Biomedical Data Fusion: A Review. Brief Bioinform. 2022;23(2). doi:10.1093/bib/bbab569
Keskinbora K, Güven F. Artificial Intelligence and Ophthalmology. Turk J Ophthalmol. 2020;50(1):37-43. doi:10.4274/tjo.galenos.2020.78989
Manimegalai P, Suresh Kumar R, Valsalan P, Dhanagopal R, Vasanth Raj PT, Christhudass J. 3D Convolutional Neural Network Framework with Deep Learning for Nuclear Medicine. Scanning. 2022;2022:1-9. doi:10.1155/2022/9640177
Kriegeskorte N, Golan T. Neural Network Models and Deep Learning. Curr Biol. 2019;29(7):R231—R236. doi:10.1016/j.cub.2019.02.034
Coleman S, Kerr D, Zhang Y. Image Sensing and Processing with Convolutional Neural Networks. Sensors. 2022;22(10):3612. doi:10.3390/s22103612
Sumida I, Magome T, Kitamori H, et al. Deep Convolutional Neural Network for Reduction of Contrast-Enhanced Region on CT Images. J Radiat Res. 2019;60(5):586-594. doi:10.1093/jrr/rrz030
Campos V, Sastre F, Yagües M, Bellver M, Giró-i-Nieto X, Torres J. Distributed Training Strategies for a Computer Vision Deep Learning Algorithm on a Distributed GPU Cluster. Procedia Comput Sci. 2017;108:315-324. doi:10.1016/j.procs.2017.05.074
Schmidt-Erfurth U, Sadeghipour A, Gerendas BS, Waldstein SM, Bogunović H. Artificial Intelligence in Retina. Prog Retin Eye Res. 2018;67:1-29. doi:10.1016/j.preteyeres.2018.07.004
Khan SM, Liu X, Nath S i in. A Global Review of Publicly Available Datasets for Ophthalmological Imaging: Barriers to Access, Usability, and Generalisability. Lancet Digit Health. 2021;3(1):e51-e66. doi:10.1016/s2589-7500(20)30240-5
Grzybowski A, Brona P. Analysis and Comparison of two Artificial Intelligence Diabetic Retinopathy Screening Algorithms in a Pilot Study: IDx-DR and Retinalyze. J Clin Med. 2021;10(11):2352. doi:10.3390/jcm10112352
Ribeiro L, Oliveira CM, Neves C, Ramos JD, Ferreira H, Cunha-Vaz J. Screening for Diabetic Retinopathy in the Central Region of Portugal. Added Value of Automated ‘disease/no disease' Grading. Ophthalmologica. 2014;233(2):96-103. doi:10.1159/000368426
Ribeiro ML, Nunes SG, Cunha-Vaz JG. Microaneurysm Turnover at the Macula Predicts Risk of Development of Clinically Significant Macular Edema in Persons with Mild Nonproliferative Diabetic Retinopathy. Diabetes Care. 2012;36(5):1254-1259. doi:10.2337/dc12-1491
Pappuru RK, Ribeiro L, Lobo C, Alves D, Cunha-Vaz J. Microaneurysm Turnover is a Predictor of Diabetic Retinopathy Progression. Br J Ophthalmol. 2018;103(2):222-226. doi:10.1136/bjophthalmol-2018-311887
Haritoglou C, Kernt M, Neubauer A, et al. Microaneurysm Formation Rate as a Predictive Marker for Progression to Clinically Significant Macular Edema in Nonproliferative Diabetic Retinopathy. Retina. 2014;34(1):157-164. doi:10.1097/iae.0b013e318295f6de
Tufail A, Kapetanakis VV, Salas-Vega S, et al. An Observational Study to Assess if Automated Diabetic Retinopathy Image Assessment Software can Replace One or More Steps of Manual Imaging Grading and to Determine Their Cost-Effectiveness. Health Technol Assess. 2016;20(92):1-72. doi:10.3310/hta20920
Rajalakshmi R, Subashini R, Anjana RM, Mohan V. Automated Diabetic Retinopathy Detection in Smartphone-Based Fundus Photography Using Artificial Intelligence. Eye. 2018;32(6):1138-1144. doi:10.1038/s41433-018-0064-9
Gulshan V, Peng L, Coram M, et al. Development and Validation of a Deep Learning Algorithm for Detection of Diabetic Retinopathy in Retinal Fundus Photographs. JAMA. 2016;316(22):2402. doi:10.1001/jama.2016.17216
Ruamviboonsuk P, Krause J, Chotcomwongse P, et al. Deep Learning Versus Human Graders for Classifying Diabetic Retinopathy Severity in a Nationwide Screening Program. NPJ Digit Med. 2019;2(1). doi:10.1038/s41746-019-0099-8
Ting DS, Cheung CY, Lim G, et al. Development and Validation of a Deep Learning System for Diabetic Retinopathy and Related Eye Diseases Using Retinal Images From Multiethnic Populations With Diabetes. JAMA. 2017;318(22):2211. doi:10.1001/jama.2017.18152
Bawankar P, Shanbhag N, K SS, et al. Sensitivity and Specificity of Automated Analysis of Single-Field Non-Mydriatic Fundus Photographs by Bosch DR Algorithm—Comparison with Mydriatic Fundus Photography (ETDRS) for Screening in Undiagnosed Diabetic Retinopathy. PLOS ONE. 2017;12(12):e0189854. doi:10.1371/journal.pone.0189854
Larsen N, Godt J, Grunkin M, Lund-Andersen H, Larsen M. Automated Detection of Diabetic Retinopathy in a Fundus Photographic Screening Population. Investig Opthalmology Amp Vis Sci. 2003;44(2):767. doi:10.1167/iovs.02-0417
Hansen AB, Hartvig NV, Jensen MS, Borch-Johnsen K, Lund-Andersen H, Larsen M. Diabetic Retinopathy Screening Using Digital Non-Mydriatic Fundus Photography and Automated Image Analysis. Acta Ophthalmol Scand. 2004;82(6):666-672. doi:10.1111/j.1600-0420.2004.00350.x
Larsen M, Godt J, Larsen N, et al. Automated Detection of Fundus Photographic Red Lesions in Diabetic Retinopathy. Investig Opthalmology Amp Vis Sci. 2003;44(2):761. doi:10.1167/iovs.02-0418
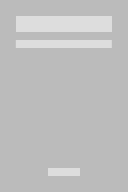
Opublikowane
Licencja

Utwór dostępny jest na licencji Creative Commons Uznanie autorstwa – Użycie niekomercyjne – Bez utworów zależnych 4.0 Międzynarodowe.