Sztuczna inteligencja w projektowaniu nowych leków oraz w repozycjonowaniu już istniejących
Słowa kluczowe:
sztuczna inteligencja, uczenie maszynowe, farmakologia, repozycjonowanie lekuStreszczenie
W ostatnich latach, efektywność procesu projektowania oraz produkowania nowych leków posiada tendencję spadkową. Zwiększająca się cena, potrzebny czas, oraz nakład wymaganej pracy wymagane do powstania nowego leku, obrały niemożliwy do utrzymania w przyszłości trend. Zmianę na korzyść w tym obszarze nauki, może przynieść wprowadzenie do standardu w procesie powstawania leków, technik wykorzystujących sztuczną inteligencję (AI), machine learning (ML), deep learning (DL), oraz zastosowanie repozycjonowania leków (ang. drug repurposing), czyli procesu wykorzystującego AI w przeanalizowaniu oraz ewentualnym zmodyfikowaniu już istniejących leków, w celu wykorzystania ich w innych lub nowych chorobach, co zostało dokonane na przykład w onkologii, lub podczas pandemii COVID-19, która wymagała szybkiego znalezienia leków pomocnych w zwalczaniu tej mało rozumianej wówczas choroby. Póki co AI nie jest jeszcze w stanie w pełni objąć różnorodnego oraz rozległego systemu danych biologicznych, w wyniku czego nie powstały jeszcze leki, których proces powstawania opierałby się wyłącznie na technikach z wykorzystaniem AI. Mimo tych ograniczeń, powstało już wiele działających systemów, które okazały się obiecujące i które przynoszą duże nadzieje na postęp w tej dziedzinie farmakologii. Celem tego artykułu jest przybliżenie procesów towarzyszących powstawaniu leków, oraz sposobów wykorzystania AI w celu ich usprawnienia. Artykuł ten również opisze procesy ponownego zastosowania już istniejących leków, które zostały z powodzeniem zastosowane np. podczas pandemii COVID-19.
Bibliografia
Drews J. Drug Discovery: A Historical Perspective. Science. 2000;287(5460):1960-1964. doi:10.1126/science.287.5460.1960
Aminov RI. A Brief History of the Antibiotic Era: Lessons Learned and Challenges for the Future. Front Microbio. 2010;1. doi:10.3389/fmicb.2010.00134
Fleming, A. (1941). Penicillin. British medical journal, 2(4210), 386.
Hare R. New light on the history of penicillin. Med Hist. 1982;26(1):1-24. doi:10.1017/s0025727300040758
Emerging Applications of Metabolomics in Drug Development. J Metabol Syst Biol. 2018;3(1):01-03. doi:10.13188/2329-1583.1000007
Park JW, Kerbel RS, Kelloff GJ, i in. Rationale for Biomarkers and Surrogate End Points in Mechanism-Driven Oncology Drug Development. Clinical Cancer Research. 2004;10(11):3885-3896. doi:10.1158/1078-0432.ccr-03-0785
Analiza Procesów Kształtowania Cen Leków Na Rynku Farmaceutycznym W Polsce. Część 1 the Analysis of the Medicines Price Formation Processes on the Pharmaceutical Market in Poland. Part 1. Accessed April 16, 2023. https://dbc.wroc.pl/Content/30551/PDF/46.pdf
Deore AB, Dhumane JR, Wagh R, Sonawane R. The Stages of Drug Discovery and Development Process. Asian J Pharm Res Dev. 2019;7(6):62-67. doi:10.22270/ajprd.v7i6.616
Żołnierek, J., Nurzyński, P., & Rzepecki, P. (2007). Nowe leki stosowane w leczeniu przerzutowego raka nerki. Współcz Onkol, 11, 41-47.
Makridakis S. The forthcoming Artificial Intelligence (AI) revolution: Its impact on society and firms. Futures. 2017;90:46-60. doi:10.1016/j.futures.2017.03.006
Bishop CM. Model-based machine learning. Phil Trans R Soc A. 2013;371(1984):20120222. doi:10.1098/rsta.2012.0222
VoPham T, Hart JE, Laden F, Chiang YY. Emerging trends in geospatial artificial intelligence (geoAI): potential applications for environmental epidemiology. Environ Health. 2018;17(1). doi:10.1186/s12940-018-0386-x
Lee JG, Jun S, Cho YW, i in. Deep Learning in Medical Imaging: General Overview. Korean J Radiol. 2017;18(4):570. doi:10.3348/kjr.2017.18.4.570
Gunčar G, Kukar M, Notar M, i in. An application of machine learning to haematological diagnosis. Sci Rep. 2018;8(1). doi:10.1038/s41598-017-18564-8
Koohy H. The rise and fall of machine learning methods in biomedical research. F1000Res. 2018;6:2012. doi:10.12688/f1000research.13016.2
Young JD, Cai C, Lu X. Unsupervised deep learning reveals prognostically relevant subtypes of glioblastoma. BMC Bioinformatics. 2017;18(S11). doi:10.1186/s12859-017-1798-2
Patel L, Shukla T, Huang X, Ussery DW, Wang S. Machine Learning Methods in Drug Discovery. Molecules. 2020;25(22):5277. doi:10.3390/molecules25225277
Coronato A, Naeem M, De Pietro G, Paragliola G. Reinforcement learning for intelligent healthcare applications: A survey. Artificial Intelligence in Medicine. 2020;109:101964. doi:10.1016/j.artmed.2020.101964
Chen H, Engkvist O, Wang Y, Olivecrona M, Blaschke T. The rise of deep learning in drug discovery. Drug Discovery Today. 2018;23(6):1241-1250. doi:10.1016/j.drudis.2018.01.039
Paul D, Sanap G, Shenoy S, Kalyane D, Kalia K, Tekade RK. Artificial intelligence in drug discovery and development. Drug Discovery Today. 2021;26(1):80-93. doi:10.1016/j.drudis.2020.10.010
Gupta A, Müller AT, Huisman BJH, Fuchs JA, Schneider P, Schneider G. Generative Recurrent Networks for De Novo Drug Design. Mol Inf. 2017;37(1-2):1700111. doi:10.1002/minf.201700111
Chan YK, Chen YF, Pham T, Chang W, Hsieh MY. Artificial Intelligence in Medical Applications. Journal of Healthcare Engineering. 2018;2018:1-2. doi:10.1155/2018/4827875
Fitzer K, Haeuslschmid R, Blasini R, i in. Patient Recruitment System for Clinical Trials: Mixed Methods Study About Requirements at Ten University Hospitals. JMIR Med Inform. 2022;10(4):e28696. doi:10.2196/28696
Mamoshina P, Vieira A, Putin E, Zhavoronkov A. Applications of Deep Learning in Biomedicine. Mol Pharmaceutics. 2016;13(5):1445-1454. doi:10.1021/acs.molpharmaceut.5b00982
Xu Y, Lin K, Wang S, i in. Deep learning for molecular generation. Future Medicinal Chemistry. 2019;11(6):567-597. doi:10.4155/fmc-2018-0358
Saiakhov R, Chakravarti S, Klopman G. Effectiveness of CASE Ultra Expert System in Evaluating Adverse Effects of Drugs. Mol Inf. 2013;32(1):87-97. doi:10.1002/minf.201200081
Parca L, Pepe G, Pietrosanto M, i in. Modeling cancer drug response through drug-specific informative genes. Sci Rep. 2019;9(1). doi:10.1038/s41598-019-50720-0
Manzoni C, Kia DA, Vandrovcova J, i in. Genome, transcriptome and proteome: the rise of omics data and their integration in biomedical sciences. Briefings in Bioinformatics. 2016;19(2):286-302. doi:10.1093/bib/bbw114
Öztürk H, Özgür A, Ozkirimli E. DeepDTA: deep drug–target binding affinity prediction. Bioinformatics. 2018;34(17):i821-i829. doi:10.1093/bioinformatics/bty593
Pushpakom S, Iorio F, Eyers PA, i in. Drug repurposing: progress, challenges and recommendations. Nat Rev Drug Discov. 2018;18(1):41-58. doi:10.1038/nrd.2018.168
Gupta R, Srivastava D, Sahu M, Tiwari S, Ambasta RK, Kumar P. Artificial intelligence to deep learning: machine intelligence approach for drug discovery. Mol Divers. 2021;25(3):1315-1360. doi:10.1007/s11030-021-10217-3
Cacabelos R. Have there been improvements in Alzheimer’s disease drug discovery over the past 5 years? Expert Opinion on Drug Discovery. 2018;13(6):523-538. doi:10.1080/17460441.2018.1457645
Carracedo-Reboredo P, Liñares-Blanco J, Rodríguez-Fernández N, i in. A review on machine learning approaches and trends in drug discovery. Computational and Structural Biotechnology Journal. 2021;19:4538-4558. doi:10.1016/j.csbj.2021.08.011
Wu C, Gudivada RC, Aronow BJ, Jegga AG. Computational drug repositioning through heterogeneous network clustering. BMC Syst Biol. 2013;7(S5). doi:10.1186/1752-0509-7-s5-s6
You Y, Lai X, Pan Y, i in. Artificial intelligence in cancer target identification and drug discovery. Sig Transduct Target Ther. 2022;7(1). doi:10.1038/s41392-022-00994-0
Zheng X, Ding H, Mamitsuka H, Zhu S. Collaborative matrix factorization with multiple similarities for predicting drug-target interactions. Proceedings of the 19th ACM SIGKDD international conference on Knowledge discovery and data mining. Published online 11 sierpień 2013. doi:10.1145/2487575.2487670
Álvarez-Machancoses Ó, Fernández-Martínez JL. Using artificial intelligence methods to speed up drug discovery. Expert Opinion on Drug Discovery. 2019;14(8):769-777. doi:10.1080/17460441.2019.1621284
Macarron R, Banks MN, Bojanic D, i in. Impact of high-throughput screening in biomedical research. Nat Rev Drug Discov. 2011;10(3):188-195. doi:10.1038/nrd3368
Congreve M, Carr R, Murray C, Jhoti H. A ‘Rule of Three’ for fragment-based lead discovery? Drug Discovery Today. 2003;8(19):876-877. doi:10.1016/s1359-6446(03)02831-9
Reymond JL, van Deursen R, Blum LC, Ruddigkeit L. Chemical space as a source for new drugs. Med Chem Commun. 2010;1(1):30. doi:10.1039/c0md00020e
Reymond JL. The Chemical Space Project. Acc Chem Res. 2015;48(3):722-730. doi:10.1021/ar500432k
Mikulak-Klucznik B, Gołębiowska P, Bayly AA, i in. Computational planning of the synthesis of complex natural products. Nature. 2020;588(7836):83-88. doi:10.1038/s41586-020-2855-y
Jiménez-Luna J, Grisoni F, Weskamp N, Schneider G. Artificial intelligence in drug discovery: recent advances and future perspectives. Expert Opinion on Drug Discovery. 2021;16(9):949-959. doi:10.1080/17460441.2021.1909567
Lipinski CA, Lombardo F, Dominy BW, Feeney PJ. Experimental and computational approaches to estimate solubility and permeability in drug discovery and development settings. Advanced Drug Delivery Reviews. 2012;64:4-17. doi:10.1016/j.addr.2012.09.019
Miljković F, Rodríguez-Pérez R, Bajorath J. Impact of Artificial Intelligence on Compound Discovery, Design, and Synthesis. ACS Omega. 2021;6(49):33293-33299. doi:10.1021/acsomega.1c05512
Genheden S, Thakkar A, Chadimová V, Reymond JL, Engkvist O, Bjerrum E. AiZynthFinder: a fast, robust and flexible open-source software for retrosynthetic planning. J Cheminform. 2020;12(1). doi:10.1186/s13321-020-00472-1
Obrezanova O. Artificial intelligence for compound pharmacokinetics prediction. Current Opinion in Structural Biology. 2023;79:102546. doi:10.1016/j.sbi.2023.102546
Gayvert KM, Madhukar NS, Elemento O. A Data-Driven Approach to Predicting Successes and Failures of Clinical Trials. Cell Chemical Biology. 2016;23(10):1294-1301. doi:10.1016/j.chembiol.2016.07.023
Mayr A, Klambauer G, Unterthiner T, Hochreiter S. DeepTox: Toxicity Prediction using Deep Learning. Front Environ Sci. 2016;3. doi:10.3389/fenvs.2015.00080
Rodrigues T, Werner M, Roth J, i in. Machine intelligence decrypts β-lapachone as an allosteric 5-lipoxygenase inhibitor. Chem Sci. 2018;9(34):6899-6903. doi:10.1039/c8sc02634c
Wang X, Liu M, Zhang L, Wang Y, Li Y, Lu T. Optimizing Pharmacokinetic Property Prediction Based on Integrated Datasets and a Deep Learning Approach. J Chem Inf Model. 2020;60(10):4603-4613. doi:10.1021/acs.jcim.0c00568
Deore AB, Dhumane JR, Wagh R, Sonawane R. The Stages of Drug Discovery and Development Process. Asian J Pharm Res Dev. 2019;7(6):62-67. doi:10.22270/ajprd.v7i6.616
Harrer S, Shah P, Antony B, Hu J. Artificial Intelligence for Clinical Trial Design. Trends in Pharmacological Sciences. 2019;40(8):577-591. doi:10.1016/j.tips.2019.05.005
Bain EE, Shafner L, Walling DP, i in. Use of a Novel Artificial Intelligence Platform on Mobile Devices to Assess Dosing Compliance in a Phase 2 Clinical Trial in Subjects With Schizophrenia. JMIR Mhealth Uhealth. 2017;5(2):e18. doi:10.2196/mhealth.7030
Pushpakom S, Iorio F, Eyers PA, i in. Drug repurposing: progress, challenges and recommendations. Nat Rev Drug Discov. 2018;18(1):41-58. doi:10.1038/nrd.2018.168
Aliper A, Plis S, Artemov A, Ulloa A, Mamoshina P, Zhavoronkov A. Deep Learning Applications for Predicting Pharmacological Properties of Drugs and Drug Repurposing Using Transcriptomic Data. Mol Pharmaceutics. 2016;13(7):2524-2530. doi:10.1021/acs.molpharmaceut.6b00248
Gottlieb A, Stein GY, Ruppin E, Sharan R. PREDICT: a method for inferring novel drug indications with application to personalized medicine. Mol Syst Biol. 2011;7(1):496. doi:10.1038/msb.2011.26
Yang B, Shi J. Developing New Cancer Nanomedicines by Repurposing Old Drugs. Angew Chem Int Ed. 2020;59(49):21829-21838. doi:10.1002/anie.202004317
Kirtonia A, Gala K, Fernandes SG, i in. Repurposing of drugs: An attractive pharmacological strategy for cancer therapeutics. Seminars in Cancer Biology. 2021;68:258-278. doi:10.1016/j.semcancer.2020.04.006
Ahmed F, Soomro AM, Chethikkattuveli Salih AR, i in. A comprehensive review of artificial intelligence and network based approaches to drug repurposing in Covid-19. Biomedicine & Pharmacotherapy. 2022;153:113350. doi:10.1016/j.biopha.2022.113350
Wang M, Cao R, Zhang L, i in. Remdesivir and chloroquine effectively inhibit the recently emerged novel coronavirus (2019-nCoV) in vitro. Cell Res. 2020;30(3):269-271. doi:10.1038/s41422-020-0282-0
Guy RK, DiPaola RS, Romanelli F, Dutch RE. Rapid repurposing of drugs for COVID-19. Science. 2020;368(6493):829-830. doi:10.1126/science.abb9332
Singh TU, Parida S, Lingaraju MC, Kesavan M, Kumar D, Singh RK. Drug repurposing approach to fight COVID-19. Pharmacol Rep. 2020;72(6):1479-1508. doi:10.1007/s43440-020-00155-6
Venkatesan P. Repurposing drugs for treatment of COVID-19. The Lancet Respiratory Medicine. 2021;9(7):e63. doi:10.1016/s2213-2600(21)00270-8
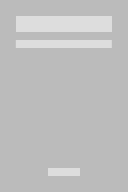
Opublikowane
Licencja

Utwór dostępny jest na licencji Creative Commons Uznanie autorstwa – Użycie niekomercyjne – Bez utworów zależnych 4.0 Międzynarodowe.