Sztuczna inteligencja w neurochirurgii – zastosowania, możliwości i zagrożenia.
Słowa kluczowe:
sztuczna inteligencja, neurochirurgia, uczenie maszynoweStreszczenie
Sztuczna inteligencja (artificial intelligence - AI) to dziedzina informatyki, która rozwija się w błyskawicznym tempie, a jej zastosowanie w medycynie, w tym w neurochirurgii, przynosi liczne korzyści. Technologie, takie jak uczenie maszynowe i sieci neuronowe, mogą pomóc w diagnozowaniu chorób OUN, planowaniu zabiegów, tworzeniu prognoz i predykcji. Dzięki precyzyjnemu planowaniu operacji i wsparciu ze strony algorytmów AI, ryzyko błędów podczas operacji może zostać zredukowane, a wyniki leczenia poprawione. Wciąż istnieją wyzwania związane z wdrożeniem tych technologii w praktykę kliniczną, w tym zagadnienia związane z bezpieczeństwem, prywatnością pacjentów i kosztami.
Bibliografia
Sengupta A, Ramaniharan AK, Gupta RK, Agarwal S, Singh A. Glioma grading using a machine-learning framework based on optimized features obtained from T1 perfusion MRI and volumes of tumor components. J Magn Reson Imaging. 2019;50(4):1295-1306. doi:10.1002/jmri.26704Pyka T, Gempt J, Hiob D, Ringel F, Schlegel J, Bette S, Wester HJ,Meyer B, Forster S (2016) Textural analysis of pre-therapeutic[18F]-FET-PET and its correlation with tumor grade and patientsurvival in high-grade gliomas. Eur J Nucl Med Mol Imaging43:133–141
Takahashi S, Takahashi W, Tanaka S, et al. Radiomics Analysis for Glioma Malignancy Evaluation Using Diffusion Kurtosis and Tensor Imaging. Int J Radiat Oncol Biol Phys. 2019;105(4):784-791. doi:10.1016/j.ijrobp.2019.07.011
Kocher M, Ruge MI, Galldiks N, Lohmann P. Applications of radiomics and machine learning for radiotherapy of malignant brain tumors. Strahlenther Onkol. 2020;196(10):856-867. doi:10.1007/s00066-020-01626-8
Qian Z, Li Y, Wang Y, et al. Differentiation of glioblastoma from solitary brain metastases using radiomic machine-learning classifiers. Cancer Lett. 2019;451:128-135. doi:10.1016/j.canlet.2019.02.054
Artzi M, Bressler I, Ben Bashat D. Differentiation between glioblastoma, brain metastasis and subtypes using radiomics analysis. J Magn Reson Imaging. 2019;50(2):519-528. doi:10.1002/jmri.26643
Hu X, Wong KK, Young GS, Guo L, Wong ST. Support vector machine multiparametric MRI identification of pseudoprogression from tumor recurrence in patients with resected glioblastoma. J Magn Reson Imaging. 2011;33(2):296-305. doi:10.1002/jmri.22432
Morey JR, Zhang X, Yaeger KA, et al. Real-World Experience with Artificial Intelligence-Based Triage in Transferred Large Vessel Occlusion Stroke Patients. Cerebrovasc Dis. 2021;50(4):450-455. doi:10.1159/000515320
Shimada Y, Tanimoto T, Nishimori M, et al. Incidental cerebral aneurysms detected by a computer-assisted detection system based on artificial intelligence: A case series. Medicine (Baltimore). 2020;99(43):e21518. doi:10.1097/MD.0000000000021518
Zhu W, Li W, Tian Z, et al. Stability Assessment of Intracranial Aneurysms Using Machine Learning Based on Clinical and Morphological Features. Transl Stroke Res. 2020;11(6):1287-1295. doi:10.1007/s12975-020-00811-2
Rava RA, Seymour SE, LaQue ME, et al. Assessment of an Artificial Intelligence Algorithm for Detection of Intracranial Hemorrhage. World Neurosurg. 2021;150:e209-e217. doi:10.1016/j.wneu.2021.02.134
Nawabi J, Kniep H, Kabiri R, et al. Neoplastic and Non-neoplastic Acute Intracerebral Hemorrhage in CT Brain Scans: Machine Learning-Based Prediction Using Radiomic Image Features [published correction appears in Front Neurol. 2021 May 21;12:687610]. Front Neurol. 2020;11:285. Published 2020 May 5. doi:10.3389/fneur.2020.00285
Teng L, Ren Q, Zhang P, Wu Z, Guo W, Ren T. Artificial Intelligence Can Effectively Predict Early Hematoma Expansion of Intracerebral Hemorrhage Analyzing Noncontrast Computed Tomography Image. Front Aging Neurosci. 2021;13:632138. Published 2021 May 26. doi:10.3389/fnagi.2021.632138
Jumah F, Raju B, Nagaraj A, et al. Uncharted Waters of Machine and Deep Learning for Surgical Phase Recognition in Neurosurgery. World Neurosurg. 2022;160:4-12. doi:10.1016/j.wneu.2022.01.020
Dundar TT, Yurtsever I, Pehlivanoglu MK, et al. Machine Learning-Based Surgical Planning for Neurosurgery: Artificial Intelligent Approaches to the Cranium. Front Surg. 2022;9:863633. Published 2022 Apr 29. doi:10.3389/fsurg.2022.863633
Zhuge Y, Krauze AV, Ning H, et al. Brain tumor segmentation using holistically nested neural networks in MRI images. Med Phys. 2017;44(10):5234-5243. doi:10.1002/mp.12481
Lang S, Hoelter P, Schmidt M, et al. Evaluation of an Artificial Intelligence-Based 3D-Angiography for Visualization of Cerebral Vasculature. Clin Neuroradiol. 2020;30(4):705-712. doi:10.1007/s00062-019-00836-7
Agarwal N, Aabedi AA, Torres-Espin A, et al. Decision tree-based machine learning analysis of intraoperative vasopressor use to optimize neurological improvement in acute spinal cord injury. Neurosurg Focus. 2022;52(4):E9. doi:10.3171/2022.1.FOCUS21743
van Niftrik CHB, van der Wouden F, Staartjes VE, et al. Machine Learning Algorithm Identifies Patients at High Risk for Early Complications After Intracranial Tumor Surgery: Registry-Based Cohort Study. Neurosurgery. 2019;85(4):E756-E764. doi:10.1093/neuros/nyz145
Durand WM, DePasse JM, Daniels AH. Predictive Modeling for Blood Transfusion After Adult Spinal Deformity Surgery: A Tree-Based Machine Learning Approach. Spine (Phila Pa 1976). 2018;43(15):1058-1066. doi:10.1097/BRS.0000000000002515
Asadi H, Dowling R, Yan B, Mitchell P. Machine learning for outcome prediction of acute ischemic stroke post intra-arterial therapy. PLoS One. 2014;9(2):e88225. Published 2014 Feb 10. doi:10.1371/journal.pone.0088225
Jabal MS, Joly O, Kallmes D, et al. Interpretable Machine Learning Modeling for Ischemic Stroke Outcome Prediction. Front Neurol. 2022;13:884693. Published 2022 May 19. doi:10.3389/fneur.2022.884693
Trevisi G, Caccavella VM, Scerrati A, et al. Machine learning model prediction of 6-month functional outcome in elderly patients with intracerebral hemorrhage. Neurosurg Rev. 2022;45(4):2857-2867. doi:10.1007/s10143-022-01802-7
Hall AN, Weaver B, Liotta E, et al. Identifying Modifiable Predictors of Patient Outcomes After Intracerebral Hemorrhage with Machine Learning. Neurocrit Care. 2021;34(1):73-84. doi:10.1007/s12028-020-00982-8
Wang R, Zeng X, Long Y, et al. Prediction of Mortality in Geriatric Traumatic Brain Injury Patients Using Machine Learning Algorithms. Brain Sci. 2023;13(1):94. Published 2023 Jan 3. doi:10.3390/brainsci13010094
Wolff J, Pauling J, Keck A, Baumbach J. The Economic Impact of Artificial Intelligence in Health Care: Systematic Review. J Med Internet Res 2020;22(2):e16866. doi: 10.2196/16866
Staartjes, V.E., Stumpo, V., Kernbach, J.M. et al. Machine learning in neurosurgery: a global survey. Acta Neurochir 162, 3081–3091 (2020). https://doi.org/10.1007/s00701-020-04532-1
Panesar SS, Kliot M, Parrish R, Fernandez-Miranda J, Cagle Y, Britz GW. Promises and Perils of Artificial Intelligence in Neurosurgery. Neurosurgery. 2020;87(1):33-44. doi:10.1093/neuros/nyz471
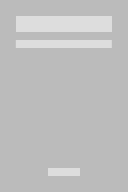
Zapowiedzi
Licencja

Utwór dostępny jest na licencji Creative Commons Uznanie autorstwa – Użycie niekomercyjne – Bez utworów zależnych 4.0 Międzynarodowe.