Implementacja technik uczenia maszynowego w przewidywaniu powodzenia ekstubacji u pacjentów wentylowanych mechanicznie
Słowa kluczowe:
uczenie maszynowe, ekstubacja, niepowodzenie ekstubacjiStreszczenie
W praktyce klinicznej istnieje wiele wyzwań, które wpływają na procesy diagnostyczne i terapeutyczne. Ze względu na złożoność procedur, poszukiwane są coraz to nowe rozwiązania, które mogłyby przynieść wymierne korzyści i odciążyć personel medyczny. W obliczu dynamicznego wzrostu zainteresowania technologiami informatycznymi, w szczególności metodami sztucznej inteligencji, rośnie także potencjał ich zastosowania w wielu dziedzinach techniki i nauki. Działem, w którym pojawia się coraz więcej publikacji o implementacji technik uczenia maszynowego (ML, ang. machine learning) jest intensywna terapia. W opiece nad pacjentem krytycznie chorym, ekstubacja jest momentem, który niesie ze sobą ryzyko groźnych powikłań i rzutuje na śmiertelność. Jednocześnie predykcja jej powodzenia uwzględnia wiele czynników, przez co określenie właściwego jej momentu przysparza wiele trudności. Celem niniejszego opracowania jest przedstawienie szans płynących z zastosowania metod ML w zwiększeniu skuteczności przewidywania sukcesu ekstubacji pacjentów wentylowanych mechanicznie. Autorzy przeprowadzili przegląd literatury za pośrednictwem bazy publikacji naukowych PUBMED przy zastosowaniu słów kluczowych “machine learning extubation”. Stosując wytyczne PRISMA dla przeglądów systematycznych, do pracy włączono 11 publikacji. Przeprowadzona analiza wyników wykazała, że trafność predykcji prowadzonej przez algorytmy ML jest wysoka i ich zastosowanie może korzystnie wpłynąć na zmniejszenie odsetka niepowodzeń ekstubacji. Należy jednak podkreślić, iż metody te wymagają dalszej ewolucji i ciągłego nadzoru celem zapewnienia jak najlepszego profilu bezpieczeństwa postępowania terapeutycznego.
Bibliografia
Epstein SK, Ciubotaru RL, Wong JB. Effect of Failed Extubation on the Outcome of Mechanical Ventilation. Chest. 1997;112(1):186-192. doi:10.1378/chest.112.1.186
Artime CA, Hagberg CA. Tracheal ExtubationDiscussion. Respir Care. 2014;59(6):991-1005. doi:10.4187/respcare.02926
Thille AW, Harrois A, Schortgen F, Brun-Buisson C, Brochard L. Outcomes of extubation failure in medical intensive care unit patients*. Crit Care Med. 2011;39(12):2612-2618. doi:10.1097/CCM.0b013e3182282a5a
Mitchell V, Dravid R, Patel A, Swampillai C, Higgs A. Difficult Airway Society Guidelines for the management of tracheal extubation. Anaesthesia. 2012;67(3):318-340. doi:10.1111/j.1365-2044.2012.07075.x
Cavallone LF, Vannucci A. Extubation of the Difficult Airway and Extubation Failure. Anesth Analg. 2013;116(2):368-383. doi:10.1213/ANE.0b013e31827ab572
Epstein S. Decision to extubate. Intensive Care Med. 2002;28(5):535-546. doi:10.1007/s00134-002-1268-8
Rothaar RC, Epstein SK. Extubation failure: magnitude of the problem, impact on outcomes, and prevention. Curr Opin Crit Care. 2003;9(1):59-66. doi:10.1097/00075198-200302000-00011
Al-alami AA, Zestos MM, Baraka AS. Pediatric laryngospasm: prevention and treatment. Curr Opin Anaesthesiol. 2009;22(3):388-395. doi:10.1097/ACO.0b013e32832972f3
Udeshi A, Cantie SM, Pierre E. Postobstructive pulmonary edema. J Crit Care. 2010;25(3):538.e1-538.e5. doi:10.1016/j.jcrc.2009.12.014
Guffin T, Har-el G, Sanders A. Acute postobstructive pulmonary edema. Otolaryngol Head Neck Surg . 1995;112(2):235-237.
Ellis PDM, Pallister WK. Recurrent laryngeal nerve palsy and endotracheal intubation. J Laryngol Otol. 1975;89(8):823-826. doi:10.1017/S0022215100081068
Hartley M, Vaughan R. PROBLEMS ASSOCIATED WITH TRACHEAL EXTUBATION. British Journal of Anaesthesi. 1993;71:561-568.
Hunter JM. Reversal of residual neuromuscular block: complications associated with perioperative management of muscle relaxation. Br J Anaesth. 2017;119:i53-i62. doi:10.1093/bja/aex318
Eikermann M, Vogt FM, Herbstreit F, et al. The Predisposition to Inspiratory Upper Airway Collapse during Partial Neuromuscular Blockade. Am J Respir Crit Care Med. 2007;175(1):9-15. doi:10.1164/rccm.200512-1862OC
Benham-Hermetz J, Mitchell V. Safe tracheal extubation after general anaesthesia. BJA Educ. 2021;21(12):446-454. doi:10.1016/j.bjae.2021.07.003
Cooper RM. Extubation and Changing Endotracheal Tubes. In: Benumof’s Airway Management. Elsevier; 2007:1146-1180. doi:10.1016/B978-032302233-0.50056-1
Elia S, Liu P, Chrusciel C, Hilgenberg A, Skourtis C, Lappas D. Effects of tracheal extubation on coronary blood flow, myocardial metabolism and systemic haemodynamic responses. Canadian Journal of Anaesthesia. 1989;36(1):2-8. doi:10.1007/BF03010879
Fisher MMcD, Raper RF. The “cuff-leak” test for extubation. Anaesthesia. 1992;47(1):10-12. doi:10.1111/j.1365-2044.1992.tb01943.x
Quintard H, l’Her E, Pottecher J, et al. Intubation and extubation of the ICU patient. Anaesth Crit Care Pain Med. 2017;36(5):327-341. doi:10.1016/j.accpm.2017.09.001
Boles JM, Bion J, Connors A, et al. Weaning from mechanical ventilation. European Respiratory Journal. 2007;29(5):1033-1056. doi:10.1183/09031936.00010206
Epstein SK. Weaning from ventilatory support. Curr Opin Crit Care. 2009;15(1):36-43. doi:10.1097/MCC.0b013e3283220e07
Lumb AB. Just a little oxygen to breathe as you go off to sleep…is it always a good idea? Br J Anaesth. 2007;99(6):769-771. doi:10.1093/bja/aem329
Hagberg C, Georgi R, Krier C. Complications of managing the airway. Best Pract Res Clin Anaesthesiol. 2005;19(4):641-659. doi:10.1016/j.bpa.2005.08.002
Zhao QY, Wang H, Luo JC, et al. Development and Validation of a Machine-Learning Model for Prediction of Extubation Failure in Intensive Care Units. Front Med (Lausanne). 2021;8. doi:10.3389/fmed.2021.676343
Fleuren LM, Dam TA, Tonutti M, et al. Predictors for extubation failure in COVID-19 patients using a machine learning approach. Crit Care. 2021;25(1):448. doi:10.1186/s13054-021-03864-3
Igarashi Y, Ogawa K, Nishimura K, Osawa S, Ohwada H, Yokobori S. Machine learning for predicting successful extubation in patients receiving mechanical ventilation. Front Med (Lausanne). 2022;9. doi:10.3389/fmed.2022.961252
Hur S, Min JY, Yoo J, et al. Development and Validation of Unplanned Extubation Prediction Models Using Intensive Care Unit Data: Retrospective, Comparative, Machine Learning Study. J Med Internet Res. 2021;23(8):e23508. doi:10.2196/23508
Fabregat A, Magret M, Ferré JA, et al. A Machine Learning decision-making tool for extubation in Intensive Care Unit patients. Comput Methods Programs Biomed. 2021;200:105869. doi:10.1016/j.cmpb.2020.105869
Pai KC, Su SA, Chan MC, Wu CL, Chao WC. Explainable machine learning approach to predict extubation in critically ill ventilated patients: a retrospective study in central Taiwan. BMC Anesthesiol. 2022;22(1):351. doi:10.1186/s12871-022-01888-y
Otaguro T, Tanaka H, Igarashi Y, et al. Machine Learning for Prediction of Successful Extubation of Mechanical Ventilated Patients in an Intensive Care Unit: A Retrospective Observational Study. Journal of Nippon Medical School. 2021;88(5):JNMS.2021_88-508. doi:10.1272/jnms.JNMS.2021_88-508
Xia M, Jin C, Cao S, et al. Development and validation of a machine-learning model for prediction of hypoxemia after extubation in intensive care units. Ann Transl Med. 2022;10(10):577-577. doi:10.21037/atm-22-2118
Wang H, Zhao QY, Luo JC, et al. Early prediction of noninvasive ventilation failure after extubation: development and validation of a machine-learning model. BMC Pulm Med. 2022;22(1):304. doi:10.1186/s12890-022-02096-7
Fukuchi K, Osawa I, Satake S, et al. The Contribution of Chest X-Ray to Predict Extubation Failure in Mechanically Ventilated Patients Using Machine Learning-Based Algorithms. Crit Care Explor. 2022;4(6):e0718. doi:10.1097/CCE.0000000000000718
Huang H, Wang J, Zhu Y, et al. Development of a Machine-Learning Model for Prediction of Extubation Failure in Patients with Difficult Airways after General Anesthesia of Head, Neck, and Maxillofacial Surgeries. J Clin Med. 2023;12(3):1066. doi:10.3390/jcm12031066
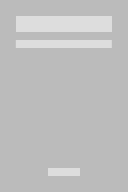
Opublikowane
Licencja

Utwór dostępny jest na licencji Creative Commons Uznanie autorstwa – Użycie niekomercyjne – Bez utworów zależnych 4.0 Międzynarodowe.