Zastosowania i możliwości wykorzystania sztucznej inteligencji w farmakologii
Słowa kluczowe:
uczenie maszynowe, sztuczna inteligencja, leki, farmakologia, farmakologia klinicznaStreszczenie
W ostatnich latach sztuczna inteligencja (ang. artificial intelligence AI) i uczenie maszynowe (ang. machine learning ML) zaczęły odgrywać coraz większą rolę w farmakologii, przyspieszając proces odkrywania oraz badania leków. W tej pracy przedstawiono przegląd najważniejszych metod i narzędzi AI/ML wykorzystywanych w farmakologii, w tym predykcję właściwości fizykochemicznych związków chemicznych, identyfikację nowych celów terapeutycznych oraz modelowanie molekularne. Omówiono zastosowanie AI/ML w procesie selekcji i priorytetyzacji nowych celów terapeutycznych, który jest kluczowym etapem w badaniach farmakologicznych. W pracy zaprezentowano także wykorzystanie AI/ML w projektowaniu i optymalizacji leków, które może prowadzić do skrócenia czasu odkrywania nowych leków oraz obniżenia kosztów badawczych. Wnioski wyciągnięte z poniższego przeglądu wskazują na to, że AI/ML mają ogromny potencjał w przyspieszeniu procesu odkrywania i rozwijania nowych leków. Pomimo pewnych wyzwań technicznych, takich jak niedostatek danych i brak standardowych protokołów badawczych, AI/ML stanowią coraz ważniejsze narzędzie w farmakologii i będą odgrywać kluczową rolę w przyszłych badaniach farmakologicznych.
Bibliografia
Ietswaart R, Arat S, Chen AX, et al. Machine learning guided association of adverse drug reactions with in vitro target-based pharmacology. EBioMedicine. 2020;57:102837. doi:https://doi.org/10.1016/j.ebiom.2020.102837
Helm JM, Swiergosz AM, Haeberle HS, et al. Machine Learning and Artificial Intelligence: Definitions, Applications, and Future Directions. Current Reviews in Musculoskeletal Medicine. 2020;13(1):69-76. doi:https://doi.org/10.1007/s12178-020-09600-8
Stulp F, Sigaud O. Many regression algorithms, one unified model: A review. Neural Networks. 2015;69:60-79. doi:https://doi.org/10.1016/j.neunet.2015.05.005
Chicco D. Ten quick tips for machine learning in computational biology. BioData Mining. 2017;10(1). doi:https://doi.org/10.1186/s13040-017-0155-3
Boulesteix AL. Ten Simple Rules for Reducing Overoptimistic Reporting in Methodological Computational Research. Lewitter F, ed. PLOS Computational Biology. 2015;11(4):e1004191. doi:https://doi.org/10.1371/journal.pcbi.1004191
Koteluk O, Wartecki A, Mazurek S, Kołodziejczak I, Mackiewicz A. How Do Machines Learn? Artificial Intelligence as a New Era in Medicine. Journal of Personalized Medicine. 2021;11(1):32. doi:https://doi.org/10.3390/jpm11010032
Altae-Tran H, Ramsundar B, Pappu AS, Pande V. Low Data Drug Discovery with One-Shot Learning. ACS Central Science. 2017;3(4):283-293. doi:https://doi.org/10.1021/acscentsci.6b00367
Libbrecht MW, Noble WS. Machine learning applications in genetics and genomics. Nature Reviews Genetics. 2015;16(6):321-332. doi:https://doi.org/10.1038/nrg3920
Jyoti B, Sharma AK. AntMiner: Bridging the Gap Between Data Mining Classification Rule Discovery and Bio-Inspired Algorithms. Proceedings of International Conference on IoT Inclusive Life (ICIIL 2019), NITTTR Chandigarh, India. Published online 2020:269-277. doi:https://doi.org/10.1007/978-981-15-3020-3_25
Alloghani M, Al-Jumeily D, Mustafina J, Hussain A, Aljaaf AJ. A Systematic Review on Supervised and Unsupervised Machine Learning Algorithms for Data Science. Unsupervised and Semi-Supervised Learning. Published online September 5, 2019:3-21. doi:https://doi.org/10.1007/978-3-030-22475-2_1
Santos R, Ursu O, Gaulton A, et al. A comprehensive map of molecular drug targets. Nature Reviews Drug Discovery. 2016;16(1):19-34. doi:https://doi.org/10.1038/nrd.2016.230
Bang S, Kim JH, Shin H. Causality modeling for directed disease network. Bioinformatics. 2016;32(17):i437-i444. doi:https://doi.org/10.1093/bioinformatics/btw439
S. Liu C, Ruthirakuhan M, A. Chau S, Herrmann N, F. Carvalho A, L. Lanctôt K. Pharmacological Management of Agitation and Aggression in Alzheimer’s Disease: A Review of Current and Novel Treatments. Current Alzheimer Research. 2016;13(10):1134-1144. Accessed February 26, 2023. https://www.ingentaconnect.com/content/ben/car/2016/00000013/00000010/art00007
Gialeli C, Theocharis AD, Karamanos NK. Roles of matrix metalloproteinases in cancer progression and their pharmacological targeting. FEBS Journal. 2010;278(1):16-27. doi:https://doi.org/10.1111/j.1742-4658.2010.07919.x
Stulp F, Sigaud O. Many regression algorithms, one unified model: A review. Neural Networks. 2015;69:60-79. doi:https://doi.org/10.1016/j.neunet.2015.05.005
Oprea TI, Bologa CG, Brunak S, et al. Unexplored therapeutic opportunities in the human genome. Nature Reviews Drug Discovery. 2018;17(5):317-332. doi:https://doi.org/10.1038/nrd.2018.14
Edwards AM, Isserlin R, Bader GD, Frye SV, Willson TM, Yu FH. Too many roads not taken. Nature. 2011;470(7333):163-165. doi:https://doi.org/10.1038/470163a
Shen Y, Yuan K, Dai J, Tang B, Yang M, Lei K. KGDDS: A System for Drug-Drug Similarity Measure in Therapeutic Substitution based on Knowledge Graph Curation. Journal of Medical Systems. 2019;43(4). doi:https://doi.org/10.1007/s10916-019-1182-z
Sun Y, Barber R, Gupta M, Aggarwal CC, Han J. Co-author Relationship Prediction in Heterogeneous Bibliographic Networks. 2011 International Conference on Advances in Social Networks Analysis and Mining. Published online July 2011. doi:https://doi.org/10.1109/asonam.2011.112
Papadimitriou S, Gazzo A, Versbraegen N, et al. Predicting disease-causing variant combinations. Proceedings of the National Academy of Sciences. Published online May 24, 2019:201815601. doi:https://doi.org/10.1073/pnas.1815601116
Bang S, Kim JH, Shin H. Causality modeling for directed disease network. Bioinformatics. 2016;32(17):i437-i444. doi:https://doi.org/10.1093/bioinformatics/btw439
DiMasi JA, Grabowski HG, Hansen RW. Innovation in the pharmaceutical industry: New estimates of R&D costs. Journal of health economics. 2016;47(47):20-33. doi:https://doi.org/10.1016/j.jhealeco.2016.01.012
Paul D, Sanap G, Shenoy S, Kalyane D, Kalia K, Tekade RK. Artificial intelligence in drug discovery and development. Drug Discovery Today. 2020;26(1). doi:https://doi.org/10.1016/j.drudis.2020.10.010
Yang X, Wang Y, Byrne R, Schneider G, Yang S. Concepts of Artificial Intelligence for Computer-Assisted Drug Discovery. Chemical Reviews. 2019;119(18):10520-10594. doi:https://doi.org/10.1021/acs.chemrev.8b00728
Gupta R, Srivastava D, Sahu M, Tiwari S, Ambasta RK, Kumar P. Artificial intelligence to deep learning: machine intelligence approach for drug discovery. Molecular Diversity. Published online April 12, 2021:1-46. doi:https://doi.org/10.1007/s11030-021-10217-3
Yang X, Wang Y, Byrne R, Schneider G, Yang S. Concepts of Artificial Intelligence for Computer-Assisted Drug Discovery. Chemical Reviews. 2019;119(18):10520-10594. doi:https://doi.org/10.1021/acs.chemrev.8b00728
Dobchev D, Karelson M. Have artificial neural networks met expectations in drug discovery as implemented in QSAR framework? Expert Opinion on Drug Discovery. 2016;11(7):627-639. doi:https://doi.org/10.1080/17460441.2016.1186876
Kim C, Zhu V, Obeid J, Lenert L. Natural language processing and machine learning algorithm to identify brain MRI reports with acute ischemic stroke. Shawe-Taylor J, ed. PLOS ONE. 2019;14(2):e0212778. doi:https://doi.org/10.1371/journal.pone.0212778
Rifaioglu AS, Atas H, Martin MJ, Cetin-Atalay R, Atalay V, Doğan T. Recent applications of deep learning and machine intelligence on in silico drug discovery: methods, tools and databases. Briefings in Bioinformatics. 2018;20(5):1878-1912. doi:https://doi.org/10.1093/bib/bby061
Rifaioglu AS, Atas H, Martin MJ, Cetin-Atalay R, Atalay V, Doğan T. Recent applications of deep learning and machine intelligence on in silico drug discovery: methods, tools and databases. Briefings in Bioinformatics. 2018;20(5):1878-1912. doi:https://doi.org/10.1093/bib/bby061
Ewing TJA, Makino S, Skillman AG, Kuntz ID. Journal of Computer-Aided Molecular Design. 2001;15(5):411-428. doi:https://doi.org/10.1023/a:1011115820450
Friesner RA, Banks JL, Murphy RB, et al. Glide: A New Approach for Rapid, Accurate Docking and Scoring. 1. Method and Assessment of Docking Accuracy. Journal of Medicinal Chemistry. 2004;47(7):1739-1749. doi:https://doi.org/10.1021/jm0306430
Trott O, Olson AJ. AutoDock Vina: Improving the speed and accuracy of docking with a new scoring function, efficient optimization, and multithreading. Journal of Computational Chemistry. 2009;31(2):NA-NA. doi:https://doi.org/10.1002/jcc.21334
Gupta R, Srivastava D, Sahu M, Tiwari S, Ambasta RK, Kumar P. Artificial intelligence to deep learning: machine intelligence approach for drug discovery. Molecular Diversity. Published online April 12, 2021:1-46. doi:https://doi.org/10.1007/s11030-021-10217-3
Jiménez-Luna J, Cuzzolin A, Bolcato G, Sturlese M, Moro S. A Deep-Learning Approach toward Rational Molecular Docking Protocol Selection. Molecules. 2020;25(11):2487. doi:https://doi.org/10.3390/molecules25112487
Pereira JC, Caffarena ER, dos Santos CN. Boosting Docking-Based Virtual Screening with Deep Learning. Journal of Chemical Information and Modeling. 2016;56(12):2495-2506. doi:https://doi.org/10.1021/acs.jcim.6b00355
Merk D, Friedrich L, Grisoni F, Schneider G. De Novo Design of Bioactive Small Molecules by Artificial Intelligence. Molecular Informatics. 2018;37(1-2):1700153. doi:https://doi.org/10.1002/minf.201700153
Domenico A, Nicola G, Daniela T, Fulvio C, Nicola A, Orazio N. De Novo Drug Design of Targeted Chemical Libraries Based on Artificial Intelligence and Pair-Based Multiobjective Optimization. Journal of Chemical Information and Modeling. 2020;60(10):4582-4593. doi:https://doi.org/10.1021/acs.jcim.0c00517
Mouchlis VD, Afantitis A, Serra A, et al. Advances in De Novo Drug Design: From Conventional to Machine Learning Methods. International Journal of Molecular Sciences. 2021;22(4). doi:https://doi.org/10.3390/ijms22041676
Liu X, Ye K, van Vlijmen HWT, IJzerman AP, van Westen GJP. An exploration strategy improves the diversity of de novo ligands using deep reinforcement learning: a case for the adenosine A2A receptor. Journal of Cheminformatics. 2019;11(1). doi:https://doi.org/10.1186/s13321-019-0355-6
Domenico A, Nicola G, Daniela T, Fulvio C, Nicola A, Orazio N. De Novo Drug Design of Targeted Chemical Libraries Based on Artificial Intelligence and Pair-Based Multiobjective Optimization. Journal of Chemical Information and Modeling. 2020;60(10):4582-4593. doi:https://doi.org/10.1021/acs.jcim.0c00517
Maziarka Ł, Pocha A, Kaczmarczyk J, Rataj K, Danel T, Warchoł M. Mol-CycleGAN: a generative model for molecular optimization. Journal of Cheminformatics. 2020;12(1). doi:https://doi.org/10.1186/s13321-019-0404-1
Paolini GV, Shapland RHB, van Hoorn WP, Mason JS, Hopkins AL. Global mapping of pharmacological space. Nature Biotechnology. 2006;24(7):805-815. doi:https://doi.org/10.1038/nbt1228
Gottlieb A, Stein GY, Ruppin E, Sharan R. PREDICT: a method for inferring novel drug indications with application to personalized medicine. Molecular Systems Biology. 2011;7:496. doi:https://doi.org/10.1038/msb.2011.26
Xia Z, Wu LY, Zhou X, Wong ST. Semi-supervised drug-protein interaction prediction from heterogeneous biological spaces. BMC Systems Biology. 2010;4(S2). doi:https://doi.org/10.1186/1752-0509-4-s2-s6
Luo Y, Zhao X, Zhou J, et al. A network integration approach for drug-target interaction prediction and computational drug repositioning from heterogeneous information. Nature Communications. 2017;8(1). doi:https://doi.org/10.1038/s41467-017-00680-8
Luo Y, Zhao X, Zhou J, et al. A network integration approach for drug-target interaction prediction and computational drug repositioning from heterogeneous information. Nature Communications. 2017;8(1). doi:https://doi.org/10.1038/s41467-017-00680-8
Giuliani S, Silva AC, Borba JVVB, et al. Computationally-guided drug repurposing enables the discovery of kinase targets and inhibitors as new schistosomicidal agents. Briggs JM, ed. PLOS Computational Biology. 2018;14(10):e1006515. doi:https://doi.org/10.1371/journal.pcbi.1006515
Yang X, Wang Y, Byrne R, Schneider G, Yang S. Concepts of Artificial Intelligence for Computer-Assisted Drug Discovery. Chemical Reviews. 2019;119(18):10520-10594. doi:https://doi.org/10.1021/acs.chemrev.8b00728
Quazi S. Role of Artificial Intelligence and Machine Learning in Bioinformatics: Drug Discovery and Drug Repurposing. wwwpreprintsorg. Published online May 14, 2021. doi:https://doi.org/10.20944/preprints202105.0346.v1
Harrer S, Shah P, Antony B, Hu J. Artificial Intelligence for Clinical Trial Design. Trends in Pharmacological Sciences. 2019;40(8):577-591. doi:https://doi.org/10.1016/j.tips.2019.05.005
Gayvert Kaitlyn M, Madhukar Neel S, Elemento O. A Data-Driven Approach to Predicting Successes and Failures of Clinical Trials. Cell Chemical Biology. 2016;23(10):1294-1301. doi:https://doi.org/10.1016/j.chembiol.2016.07.023
Zhavoronkov A, Vanhaelen Q, Oprea TI. Will Artificial Intelligence for Drug Discovery Impact Clinical Pharmacology? Clinical Pharmacology & Therapeutics. 2020;107(4):780-785. doi:https://doi.org/10.1002/cpt.1795
Zhavoronkov A, Vanhaelen Q, Oprea TI. Will Artificial Intelligence for Drug Discovery Impact Clinical Pharmacology? Clinical Pharmacology & Therapeutics. 2020;107(4):780-785. doi:https://doi.org/10.1002/cpt.1795
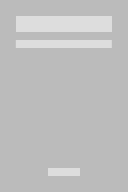
Zapowiedzi
Licencja

Utwór dostępny jest na licencji Creative Commons Uznanie autorstwa – Użycie niekomercyjne – Bez utworów zależnych 4.0 Międzynarodowe.